Harnessing the Power and Navigating the Pitfalls of Synthetic Data in AI
3 min read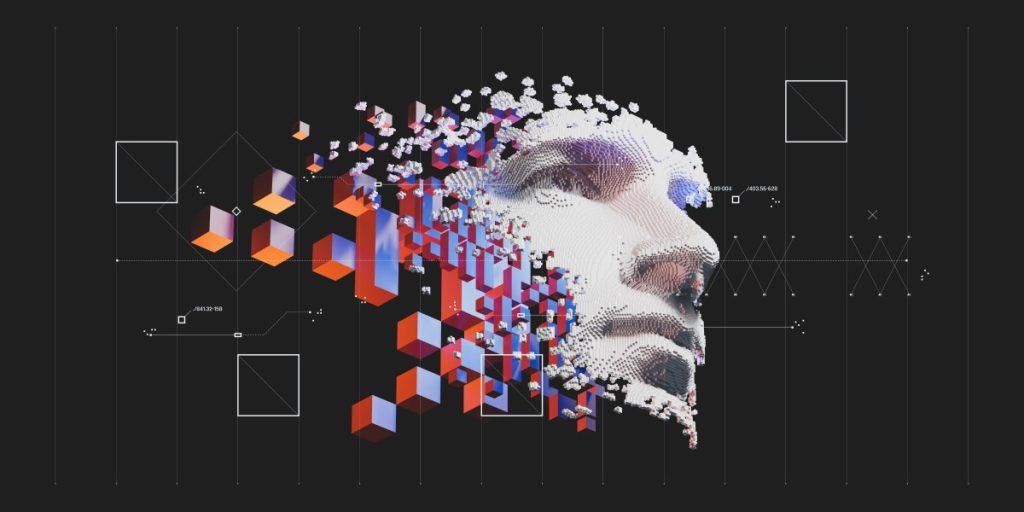
Synthetic data is revolutionizing AI development, offering new avenues for model training where real data falls short. It proves vital as original data sources become scarce and protected.
The growing trend of synthetic data utilization promises cost-effective solutions, expanding AI capabilities while raising questions about long-term effects and risks
Understanding the Role of Annotations
Annotations are the backbone of AI training. They guide AI systems in making sense of raw data. Simply put, they act as teachers for AI models, helping them recognize patterns and differentiate between various data elements. Imagine training a model with photos labeled “kitchen”; it learns to associate this label with kitchen features.
The Drying Well of Original Data
The world is facing a data scarcity crisis. Access to real, high-quality data is becoming increasingly difficult. This scarcity stems from data owners becoming protective over their assets, restricting AI companies from accessing their data. The fear of misuse and copyright issues has made them wary of opening their gates.
Synthetic Data: A Promising Solution
Synthetic data emerges as a hero in this crisis. It offers a way to create vast amounts of data without the limitations of original sources. Companies like Writer and Google are pioneering in this domain, showcasing how effective synthetic data can be in training AI models.
The cost-effectiveness of synthetic data is unmatched. For instance, creating a model like Palmyra X 004 cost just a fraction of using real data. This affordability makes it accessible even for startups with limited budgets.
Furthermore, synthetic data allows for the creation of specific scenarios that might be rare in the real world, enhancing the diversity and capability of AI models.
Risks Inherent in Synthetic Data
Despite its benefits, synthetic data isn’t foolproof. Similar to any data, its quality depends on the source. “Garbage in, garbage out” is a real threat, where poor quality input leads to unreliable AI outputs. Biased initial data could amplify errors in AI models.
Overtime, reliance solely on synthetic data may degrade model diversity. If synthetic entries lack representation, diversity issues arise. Incorporating some real data is essential to balance this. Opening up to various data sources is key in maintaining model integrity.
The Future of Data Generation
Visionaries believe synthetic data will someday be self-sustaining. However, this remains a distant dream. Present-day AI still requires human intervention to validate synthetic data, ensuring it aligns with real-world scenarios.
The marriage of real and synthetic data promises an effective future for AI. This dual approach ensures comprehensive learning, minimizing algorithmic errors. Adapting to such innovations keeps us ahead.
Combining Human Intuition and AI Innovation
While synthetic data generation techniques are evolving, the human touch remains irreplaceable. Pairing human insight with automated data generation creates synergy, optimizing AI’s learning curve. Merging these techniques mitigates risks and enhances outcomes.
The need for human oversight continues. Analyzing, evaluating, and refining synthetic data is integral. Ensuring relevance and accuracy safeguards AI from becoming biased or erroneous.
Synthetic Data’s Role in AI Development
Synthetic data bridges gaps that natural data leaves behind. It challenges boundaries, expands possibilities, and maximizes AI potential. With its strategic use, the AI landscape can be transformed, embracing previously unreachable goals.
The future of AI, driven by synthetic and real data fusion, seems bright. As we innovate, careful balance maintains AI development integrity.
A collaborative approach leverages strengths and mitigates weaknesses, preparing AI for unforeseen challenges while maximizing potential.