Understanding AI The Buzz Around Sora and Video Generation Models
4 min read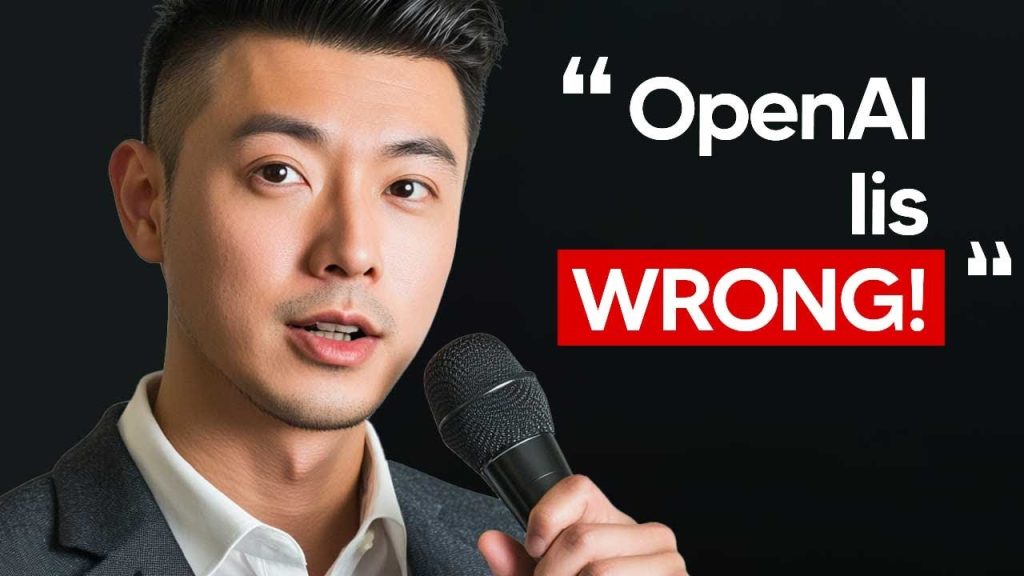
Hey there, curious minds! Today, we’re diving into the heart of a buzzing video that’s making waves online. If you’ve ever wondered how AI manages to make those mind-blowing videos, you’re in the right place.
So, what’s all the buzz? We’re talking about Sora, a video generation powerhouse that promises to revolutionize AI. Sounds exciting, right? But there’s more to it than meets the eye. Get ready to explore the ups and downs of Sora’s capabilities!
The Enigma of Sora’s Realism
Sora is undeniably impressive, creating videos so real they might trick your senses. However, there’s a catch: is Sora genuinely understanding the physics of our world? The video generation models aim to simulate reality, but are they just convincing illusions? This is where skepticism arises, pushing us to dig deeper.
Sora’s Role in Achieving AGI
OpenAI believes Sora could be pivotal in developing Artificial General Intelligence (AGI). AGI, for those new to the term, is an AI system that can perform any intellectual task a human can. But there’s a twist!
The twist lies in whether Sora’s video models can realistically simulate the physical world. While they might look promising, are they truly paving the way towards AGI? That’s the question we need to explore.
Video generation models like Sora are trained to predict future scenes. This means they try to guess what happens next in a video. They work similarly to how we imagine the next part of a movie. Yet, some experts question their accuracy in unfamiliar situations.
Inside vs. Outside the Box: The Distribution Dilemma
Sora’s models work best with familiar data. This is called ‘in distribution.’ When they encounter something new or ‘out of distribution,’ their performance drops. Imagine trying to navigate a foreign city without a map; that’s what these models face.
The concern is whether these models can generalize, or adapt to new scenarios. This ability is critical for real-world applications. Are current models falling short when stepping beyond familiar data? Critics believe so.
The Retrieval and Replay Paradox
Experts are uncovering that Sora’s video generation might be more about smart retrieval than real understanding. Think of it like memorizing instead of truly learning. This revelation poses significant challenges for achieving realistic simulations.
If these models rely on retrieving known data, it could limit their potential. They’re not creating something new; they’re piecing together existing bits. This approach could be a barrier to developing models that understand and portray real-world physics.
Understanding these models better helps us grasp the broader picture of video generation technology. The robust framework of retrieval might not be enough to meet future demands, and researchers are eager for breakthroughs.
Scaling and Synthesizing: A Path Beyond
Innovations are underway to overcome the limitations of current models. Researchers are exploring scaling and synthesizing data to enhance model versatility. This involves expanding the data pool and creating new scenarios.
While scaling data sounds like a solution, it presents challenges. More data doesn’t always equate to better understanding. Balancing quality and quantity in data is crucial.
Pioneering New Architectures
The quest for better models calls for new architectural designs. Some believe a shift towards ‘objective-driven AI’ could bring us closer to AGI. This approach focuses on planning and reasoning rather than just reacting.
Objective-driven AI uses a mental model to predict future states, mimicking human-like thinking. It adapts to changes and constraints, making it better suited for dynamic environments.
This architectural shift may redefine how we perceive AI’s potential. By focusing on strategy and adaptability, researchers hope to bridge the current gaps in AI capabilities.
Testing the Limits with VJeer
VJeer stands as a promising non-generative model. It’s designed to learn from few examples, just like humans do. This efficiency could transform AI learning processes.
Although VJeer focuses on short videos, its potential is undeniable. It prioritizes concept over pixels, ensuring effective learning without drowning in data. This innovation is a stepping stone to overcoming generalization challenges.
By refining these approaches, the AI community is inching closer to foundational changes that could redefine the landscape. VJeer’s contributions are proof of innovative strides being made.
Conclusion: A Path Forward
AI, like Sora and beyond, is a journey of constant evolution. As researchers push the boundaries, we’re witnessing advancements that inch us closer to understanding. The road to AGI is paved with curiosity and breakthroughs.
As we continue to explore AI’s capabilities, we discover both its wonders and limitations. With each step, the path to AGI becomes clearer, though challenges remain. It’s an exciting time for technology enthusiasts as innovation sparks new conversations and possibilities.