Exploring the Hidden Geometry of AI Systems
4 min read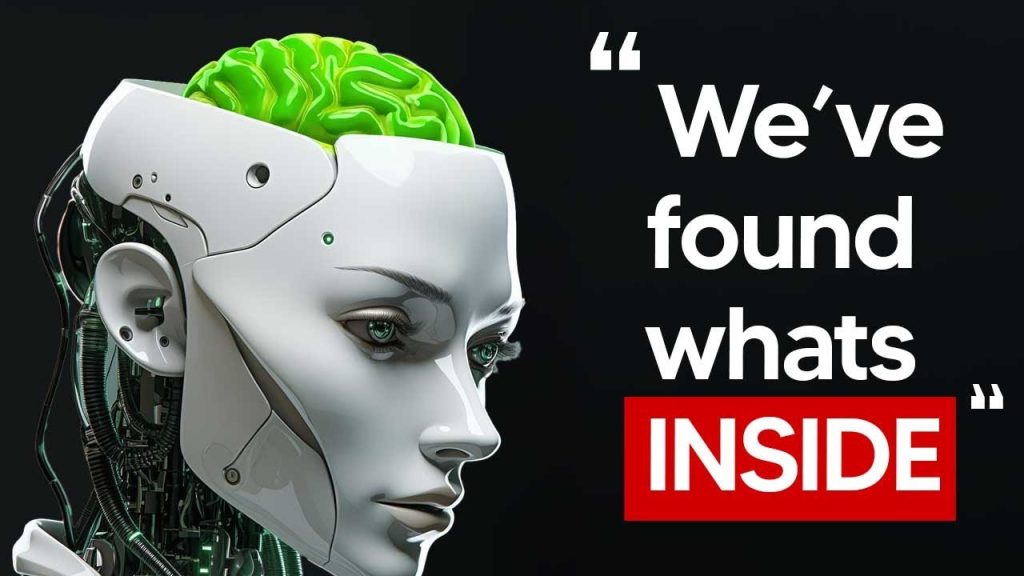
Imagine peeking under the hood of a sophisticated machine to discover its intricate workings. A new research paper unveils the captivating geometric structures of AI systems, much like delving into a realm where concepts take on a unique form. This journey promises to unravel the enigma behind how AI organizes information.
At its core, this revelation offers a fresh perspective on the mysterious world of large language models. These models, often seen as black boxes, now open up, revealing three distinct organizational levels. The structures resemble human cognition, offering a bridge between artificial and biological intelligence. Let’s dive into this fascinating exploration.
The Atomic Structure Unveiled
At the atomic level, researchers found AI organizing concepts into geometric patterns. Picture it like a 3D puzzle where related concepts connect, forming shapes. A noted example is how words like man, woman, king, and queen construct a perfect parallelogram, illustrating AI’s comprehension of gender relationships.
Brain-like Lobes
AI knowledge is arranged into distinct lobes, mirroring human brain functions. This includes the cod-sl math lobe for math and programming, a general language lobe for English text, and a dialogue lobe for conversational text. These natural structures highlight AI’s inherent ability to organize its knowledge.
When AI tackles coding or mathematical tasks, the cod-sl math lobe kicks into action. Similarly, general language tasks activate another lobe, responsible for longer texts like articles. These lobes are not programmed but emerge naturally, showing how AI systems intuitively segregate information.
Comprehending the Galaxy Structure
Exploring further, the galaxy structure of AI emerges, organizing knowledge with mathematical patterns. This layer acts as a bottleneck, retaining essential features. It efficiently compresses information, akin to how human brains process sensory inputs into manageable chunks.
By maintaining only crucial features, this structure enhances AI’s task performance. The power laws governing this organization ensure an optimal arrangement where significant components dominate, and less important ones fade, creating a hierarchy much like human information prioritization.
This pattern of efficient compression helps AI models excel across various tasks. With information distilled into high-level representations, the models focus on key aspects, discarding irrelevant details. This efficiency results in robust performance and versatility.
Implications of AI’s Inner Workings
Understanding AI’s internal organization elucidates its effectiveness in various tasks. This knowledge enables refinements in feature learning, potentially improving computational efficiency and interpretability.
Through these insights, biases can be reduced, and AI models become more transparent, crucial for sensitive applications in healthcare and finance. Furthermore, understanding these structures offers a basis for targeted enhancements, refining capabilities and reducing biases.
A Glimpse into the Future of AI
By analyzing AI structures, we edge closer to developing AI that parallels human cognitive functions. Such insights pave the way for advanced learning tools and AI-human collaboration.
These discoveries are not merely about improving AI models but also understanding intelligence itself. As these systems evolve, they could influence cognitive science and even the philosophy of the mind.
The Journey Ahead
Despite these breakthroughs, the journey to understanding AI’s structures has just begun. More research is essential to comprehend the full implications and potential improvements for AI systems.
As techniques and discoveries continue to evolve, so will our understanding of these brain-like structures. This evolving field promises breakthroughs, not just in AI design but in understanding intelligence as a whole.
With ongoing research, we stand at the cusp of a new era. These revelations, akin to unearthing secrets of the mind, might redefine our grasp of both artificial and natural intelligence.
Bridging AI and Human Cognition
Studying AI’s structures offers parallels with human cognition, suggesting universal information organization principles. This insight can help develop AI systems aligned with natural intelligence.
By drawing parallels between AI and human brains, this research provides a framework for designing future AI. Understanding these systems sheds light on both artificial and human thought processes.
The Significance of AI Research
Why does this matter? Understanding AI’s brain-like structures holds potential for AI and human cognition research. This knowledge could address cognitive impairments and aid in developing AI systems that collaborate with humans effectively.
The parallels between AI and human cognition suggest universal principles for information processing. These insights guide the design of future AI systems, making them more aligned with natural intelligence.
Moreover, this research enhances our understanding of intelligence, influencing cognitive science, neuroscience, and even the philosophy of the mind.
Embracing the Unknown
While AI structures mirror human cognition, they’re fundamentally distinct. AI operates on mathematical functions, unlike the biological processes of human brains.
AI isn’t conscious or thinking like a human. Its responses stem from learned patterns, lacking awareness. These mathematical patterns aim for computational efficiency, not replicating human brain functions.
The exploration of AI’s geometric structures bridges the gap between artificial and human intelligence. With ongoing research, we inch closer to redefining our understanding of cognition in both realms.