The Promise and Risks of Synthetic Data
4 min read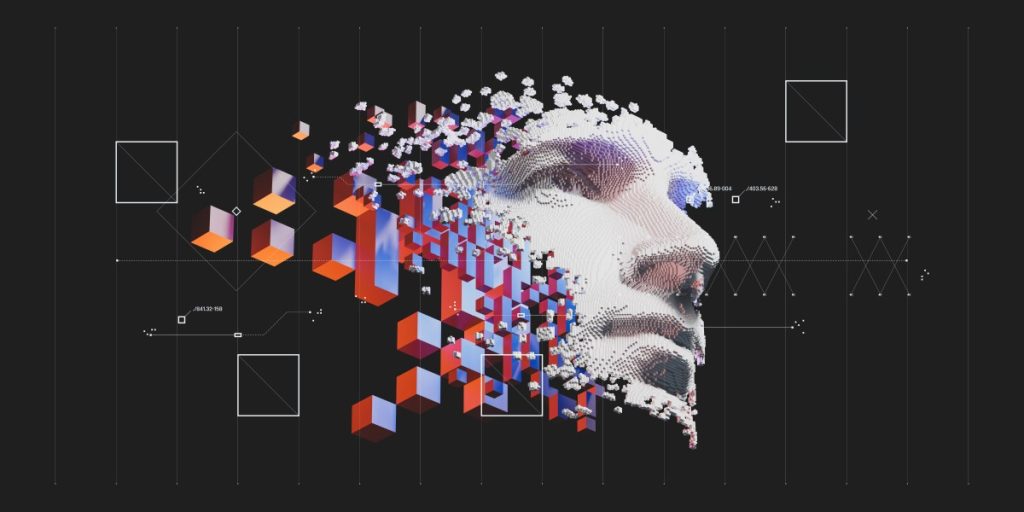
Imagine a world where machines learn from data that isn’t real but created. This is the intriguing concept of synthetic data. As real data becomes harder to find, synthetic data is gaining popularity. The journey isn’t without challenges, but the potential benefits drive innovation and progress.
AI innovation depends heavily on data, traditionally sourced from human-labeled examples. This process is resource-intensive and fraught with issues like bias and privacy concerns. Enter synthetic data—a seemingly limitless alternative promising a sustainable future for AI development. However, the path to its integration is paved with potential pitfalls. How do we navigate this?
Understanding Annotations
AI systems rely on vast examples to learn and make predictions. Annotations, or labeled data, guide these predictions, helping models distinguish objects and ideas. For instance, a model learns to identify kitchens by associating them with features like fridges, based on labeled images. Without accurate annotations, AI performance falters.
The demand for AI has skyrocketed the market for annotation services. As of now, this market is valued at $838.2 million, expected to reach $10.34 billion in 10 years. Many people globally engage in data labeling, earning money, though not always at fair rates. Sadly, workers in developing countries often earn minimal without job security.
The Data Dilemma
With rising costs and limited availability, acquiring large data sets is challenging. Public data sources are increasingly gated to protect against misuse, blocking AI model training. As a solution, developers are exploring synthetic data to maintain AI growth.
Companies and website owners restrict access to their data, fearing misuse without proper credit or compensation. This limits data for training models. Even large tech firms pay significant sums for data access. Some studies suggest critical AI development could halt between 2026-2032 if access restrictions persist.
Synthetic Data to the Rescue
Synthetic data offers a promising alternative to real-world data, overcoming limitations in data availability. It can simulate vast quantities of varied data, enabling continuous AI training and innovation.
Writer, a generative AI company, developed a model using mostly synthetic data, cutting costs to $700,000 from the millions usually needed. This success shows that synthetic data could soon dominate AI training landscapes.
Microsoft, Google, and Nvidia, among others, have embraced synthetic data for model training. This approach offers financial benefits and circumvents traditional data sourcing limitations, aligning with Gartner’s prediction that most AI data will soon be synthetic.
Challenges with Synthetic Data
However, synthetic data is not without flaws. It inherits biases and errors from the original datasets, which can result in skewed AI outputs.
A 2023 study from Rice University and Stanford highlights these risks, noting that excessive reliance on synthetic data can degrade model quality and diversity. To address this, experts suggest mixing real-world and synthetic data during training.
The creation of synthetic data isn’t a perfect science. Unintended patterns can lead to errors, affecting AI performance. Developers must carefully supervise the generation process to understand and correct potential issues.
The Business of Synthetic Data
In response to growing demand, synthetic data generation has become a lucrative industry. Estimated to be worth $2.34 billion by 2030, companies are investing in synthetic data solutions.
These companies deliver creative ways to expand limited datasets without compromising ethical or legal standards. Nvidia and Hugging Face are notable players in this space, each developing solutions to generate and utilize synthetic data effectively.
Integrating Synthetic Data Safely
To safely leverage synthetic data in AI model development, thorough review and curation are key. It cannot replace real data but can enhance it when properly integrated.
Experts emphasize examining and refining synthetic data generation. This process ensures AI models remain accurate and reliable despite integrating synthetic data.
OpenAI CEO Sam Altman envisions AI generating its own training data in the future. However, such capability is not yet realized, necessitating a blend of human oversight and synthetic data integration for now.
Future Outlook of Synthetic Data
The future of AI and synthetic data is intertwined. While synthetic data promises to address current barriers in AI development, its potential risks require careful management.
Ensuring the precision of synthetic data involves ongoing monitoring and occasional real data supplementation. This balancing act keeps AI models versatile and accurate.
With continued advancements, synthetic data stands to revolutionize AI training. However, vigilance remains paramount to preserve model integrity and innovation.
Synthetic data holds great promise for AI but requires careful handling to avoid pitfalls. By blending it with real-world data, we can harness its power effectively. The key lies in vigilance, thorough review, and continuous improvement to ensure safe AI evolution.