A New Era: Self-Driving Cars and Their Shared Intelligence
4 min read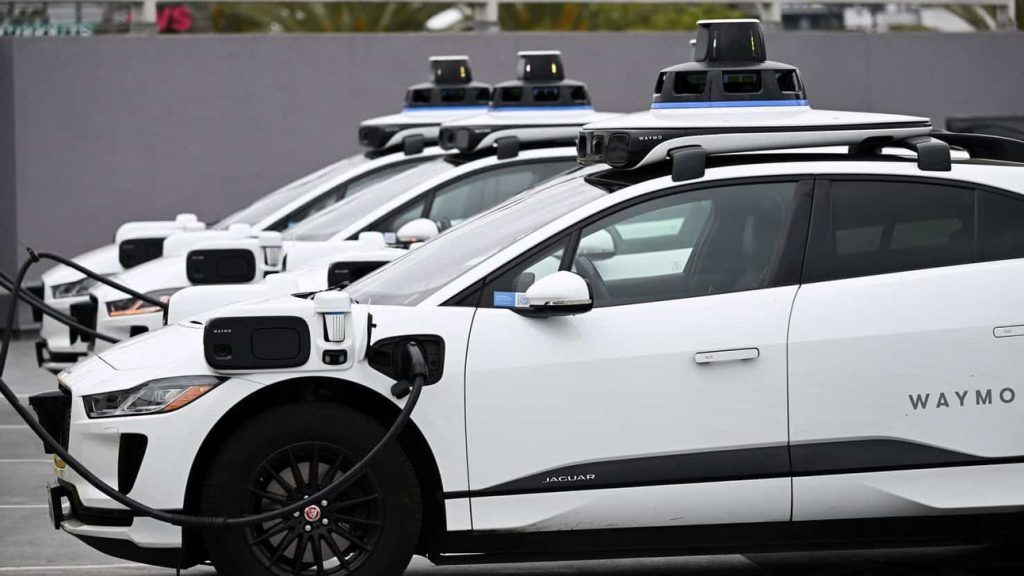
Self-driving cars are becoming smarter by the day. Researchers from NYU have designed a social network for these autonomous vehicles, enabling them to communicate efficiently. This new system, called Cached Decentralized Federated Learning, enhances the intelligence of cars by allowing them to share crucial road information.
Imagine cars that can share road conditions without even meeting. This system tackles previous communication bottlenecks, making the exchange of knowledge smoother and more efficient. With indirect model sharing, cars become aware of traffic and road signals, boosting their smart capabilities and ensuring safety.
Self-Driving Cars Join the Social Network
NYU’s Cached Decentralized Federated Learning (Cached-DFL) is a game-changer for autonomous vehicles. They can now share knowledge without direct contact, like passing gossip. Vehicles within proximity exchange AI models swiftly, keeping their data updated and relevant.
This innovative approach ensures that even if two cars never physically meet, they can still learn from each other’s experiences. It’s like a digital rumor mill that keeps cars informed and ready for any road challenges, expanding their collective wisdom.
Challenges in Autonomous Communication
Before the introduction of Cached-DFL, AVs were like strangers on the road, unable to share knowledge efficiently. This resulted in limited learning and adaptability.
However, Cached-DFL changes this by allowing autonomous vehicles to relay information through a chain of shared experiences. This indirect communication ensures cars are always learning and adapting to their environment.
Such a system significantly enhances the capability of AVs, making them more efficient in navigating diverse traffic patterns. The ability to share data without a central server also means faster processing and less latency.
The Importance of Data Privacy
Data privacy remains a top priority with Cached-DFL. Cars retain control of their data while sharing models to improve self-driving capabilities.
This decentralized method addresses concerns related to centralized data processing and privacy issues. By keeping the data exchange limited to necessary information, privacy breaches are minimized.
Thus, autonomous vehicles become not only smarter but also more secure, ensuring user trust and safety on the roads. The trust factor plays a critical role in wider adoption of self-driving technology.
Beyond Just Cars
The impact of Cached-DFL isn’t limited to just cars. Drones and other robotic systems can benefit from this learning method too.
Real-time learning allows all autonomous systems to adapt quickly to new situations. The decentralized approach aids in scalability, making it applicable to various autonomous technologies.
Hence, the broader scope of Cached-DFL could revolutionize how different intelligent systems function, increasing their efficiency in real-world scenarios.
A Glimpse Into the Future
With real-time model sharing, the future of self-driving cars looks promising. They’ll be able to adapt faster to changing road conditions.
This advancement not only boosts current capabilities but also prepares AVs for challenges they might face as technology evolves.
The continuous evolution in communication methods will see these cars becoming a regular feature on global roads, making driving safer and smarter.
The Core Mechanics of Cached-DFL
Cached-DFL relies on device-to-device communication, where cars exchange models every two minutes.
The system includes a cache of ten external models, updated frequently. This ensures that cars are never outdated, always ready for new road challenges.
This breakthrough in communication technology signifies a leap towards smarter, more reliable autonomous systems.
Benefits and Advantages
The benefits of Cached-DFL extend beyond just enhanced learning. Its decentralized nature boosts scalability and adaptability.
The technology ensures that each car can autonomously adapt while still contributing to the collective intelligence of the network.
This collaborative learning approach is set to redefine how autonomous technologies operate in the future.
Addressing Potential Concerns
While the benefits are immense, potential challenges include maintaining data privacy and managing data volume.
Efficient data management and privacy control mechanisms are essential for the seamless functioning of the system.
Despite these challenges, the overall advantages significantly outweigh the potential pitfalls, ensuring a smooth transition to smarter roads.
The Role of Artificial Intelligence
Artificial Intelligence is at the heart of Cached-DFL. It enables the decentralized learning process and model sharing among cars.
AI not only facilitates smoother communication but also ensures data accuracy and relevancy, key factors in successful autonomous navigation.
As AI technology advances, the precision and efficiency of self-driving cars will only improve, closing gaps in current autonomous systems.
Looking Ahead
The journey of self-driving cars and their shared intelligence is only beginning. With continuous innovation, they hold the potential to transform urban mobility.
The collaboration between AI, autonomous vehicles, and innovative learning methods like Cached-DFL will shape the future of transportation.
The future of autonomous vehicles is bright. With innovations like Cached-DFL, self-driving cars become safer, smarter, and more efficient. This collaborative learning is set to redefine transportation, making roads safer for everyone.