Accelerating Your AI Plans with a Strong Data Foundation
4 min read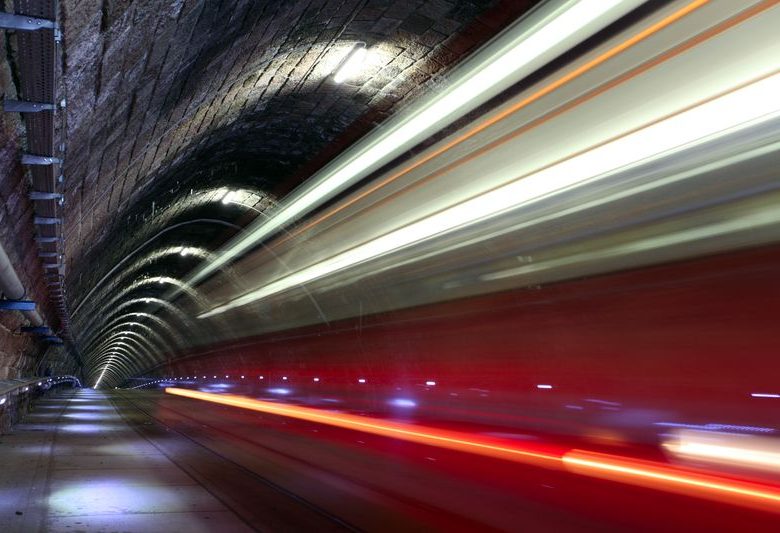
Imagine a world where artificial intelligence (AI) doesn’t just perform tasks but transforms entire businesses. Building AI systems requires more than just task-oriented learning from data. It necessitates a strong data foundation that can support and scale as the organization grows. From predictive capabilities to automated processes, AI demands a robust infrastructure. And it’s not just about having the right technology—there’s a human element too.
As more data comes in, it’s vital to have systems that can manage and extract value from it efficiently. The Chief Data Officer (CDO) of a global enterprise spanning 170 countries sheds light on how data foundation and architecture play a crucial role. With increasing data volumes, relying on AI’s cognitive capabilities becomes essential. But the real story here is how camaraderie among data professionals drives best practices and innovations. Let’s dive into how these elements come together to form the backbone of modern AI enterprises.
Accelerating AI with a Strong Data Foundation
Building artificial intelligence (AI) systems requires more than just learning to perform specific tasks from data. It necessitates a strong data foundation and infrastructure. This foundation aids organizations of all sizes as they climb the Ladder to AI.
As the Chief Data Officer (CDO) of a global enterprise spanning 170 countries, data foundation and architecture are key priorities. With the increasing data volumes, the reliance on the predictive, automated, and cognitive capabilities of AI naturally follows. This dependency helps manage and extract maximum value from vast data volumes.
The Importance of Camaraderie Among CDOs
A unique aspect of the CDO discipline is the camaraderie not only within an organization but with peers across different sectors. There’s a significant yearning within this circle of CxOs to learn from those excelling in certain areas. This inclination towards best practices is a collective response to manage and mine the valuable resource called data.
This attitude is evident at bi-annual CDO Summits, which are filled with discussions, presentations, and meetings focused on managing, exploiting, and securing data. With this spirit in mind, the AI Enterprise Accelerator was founded to build on IBM’s AI transformation.
Introducing the AI Enterprise Accelerator
The AI Enterprise Accelerator is a collaborative initiative designed to help data leaders quickly adopt solutions and processes that have driven successes at IBM. It melds three critical components: feedback from Chief Data Officers, IBM’s technology and data expertise, and IBM’s extensive AI investments.
This new service offers foundational models for clients to replicate. These models serve as examples of where AI-transformed business processes can generate value, focusing on five key topics: data strategy, AI enterprise data architecture, automated metadata generation, data privacy, and AI applications.
Data Strategy: Aligning with Business Goals
Data strategy begins with understanding the business strategy. It’s essential to create a data strategy aligning AI and data resources to the business objectives. This strategy acts as a compass for all future data-driven AI initiatives.
AI Enterprise Data Architecture: Multi-Cloud Integration
AI Enterprise Data Architecture illustrates a multi-cloud architecture and workflows. This setup allows for seamless integration and movement of data across AI and analytic workloads. It forms the bedrock of an AI enterprise.
The integration ensures that data is accessible and can move fluidly between different cloud environments, enhancing overall efficiency. This architecture supports various AI and analytics tasks, making the enterprise more agile.
Automated Metadata Generation: Adding Depth to Data
Automating metadata generation involves using deep learning and natural language understanding. This approach adds significant value by making data more accessible and useful.
The technical system specifications and business process workflows needed for automated metadata generation ensure that data is well-organized and easily searchable.
This automation not only saves time but also enhances data accuracy and usability across different applications. It becomes easier to manage massive data volumes effectively.
Data Privacy: Meeting Regulatory Demands
Data privacy is a critical aspect of deploying AI resources. The approach includes frameworks for governance, security, communication, and automation to comply with regulations like GDPR.
These frameworks not only help in meeting current data privacy regulations but also prepare organizations for future compliance requirements. They provide a structured way to ensure data security and privacy.
AI Applications: Diverse and Impactful Uses
AI applications are diverse, covering areas like sales enablement, contractual insights, and risk insights. For instance, AI-powered seller recommendations can provide a comprehensive view of clients, enhancing sales strategies.
Another example is using AI to manage contractual relationships by identifying opportunities for expansion. This proactive approach helps in maintaining and growing client relationships.
AI can also be used to assess the impact of natural disasters on data centers in real-time. This capability helps in risk management and ensures business continuity.
Expanding AI Capabilities Through IBM Resources
The AI Enterprise Accelerator leverages IBM resources such as IBM Analytics University, IBM Design Thinking Workshops, and IBM Cloud and Cognitive Garages. These resources enable clients to replicate the capabilities discussed.
By utilizing these resources, organizations can adopt AI initiatives more efficiently and effectively. This support structure is crucial for driving successful AI transformations.
In conclusion, building a strong data foundation is pivotal for scaling AI capabilities. From data strategy to AI applications, each component plays a crucial role. The AI Enterprise Accelerator shows how unifying technology, best practices, and expert insights can drive profound transformations in data management and AI implementation.
The collaboration among Chief Data Officers and IBM’s extensive resources underscore a collective commitment to excellence. As data volumes grow, the necessity for robust, scalable infrastructure becomes even more essential. The future of AI is bright, and well-architected data strategies will light the way.