Apple’s New Study A Paradigm Shift in AI Reasoning
3 min read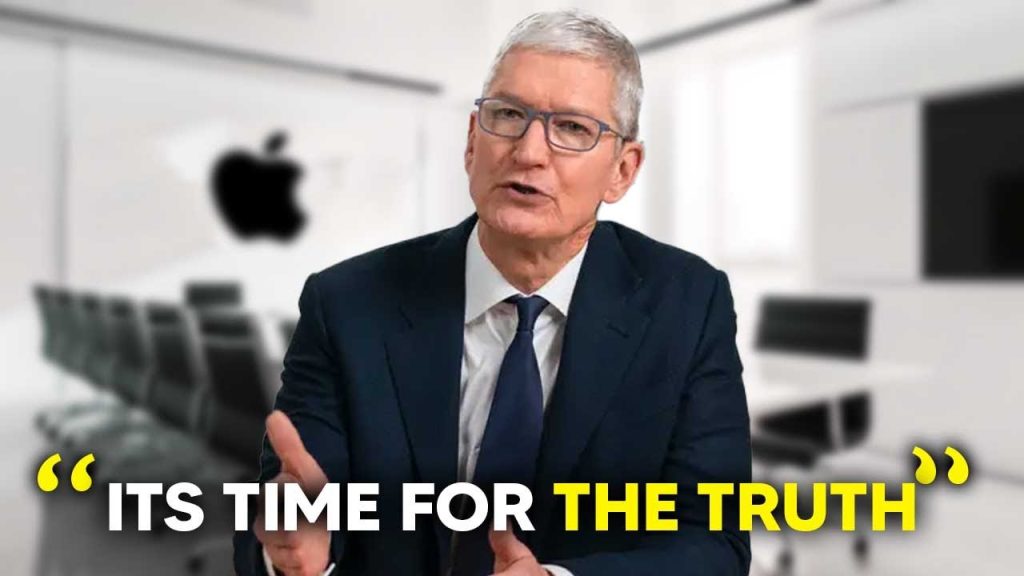
Apple’s new research paper has sparked intense discussions in the AI community.
The study reveals potential flaws in AI logic, questioning the true intelligence of current models.
Apple’s Bold Research Move
Apple’s recent research paper on AI has stirred the pot in the tech world. The document challenges the current understanding of AI models, suggesting they lack true logical reasoning. Many see this as a groundbreaking revelation that could change the future of AI development.
Most AI models, it argues, merely mimic reasoning steps from their training data. This raises questions about the genuine intelligence of models like GPT-3 or GPT-4, which were thought to be ahead of their time. The research suggests they might only be expert pattern matchers.
Apple’s study uses benchmarks like the GSM 8K to measure AI’s reasoning ability. It notes an improvement in test scores, but questions whether this is true progress or just smarter data handling. The implications could be huge for AI advancement.
Benchmarking Breakthrough or Just Noise?
The GSM 8K is used to check how smart AI models really are. Older models scored low, while today’s models hit high numbers. Apple’s research questions if these numbers truly reflect progress or if data contamination plays a role.
Data contamination can inflate scores by slipping test data into training sets. Apple created a new benchmark, GSM Symbolic, to test genuine mathematical reasoning without contamination. The results were surprising, showing variances between claimed and actual scores.
The GSM Symbolic Experiment
Apple altered names and values in math problems to test AI models. If models truly understood reasoning, changes shouldn’t affect performance. However, discrepancies between expected and actual results were significant.
Many models scored lower on GSM Symbolic than on GSM 8K. This suggests that changes to names and values confounded the AI, pointing to pattern recognition rather than true understanding. The fragility of AI reasoning is evident.
The experiments further altered problem difficulty, but results varied. While some models handled added complexity, others faltered. The inconsistency raises questions about AI’s capability in real-world problem-solving.
Irrelevant Information Causes Chaos
Adding irrelevant details to questions showed AI models’ difficulty in ignoring unnecessary information. For example, a problem about counting fruit confused models when irrelevant details were added. Where logic should prevail, models instead stumbled.
The performance drop when irrelevant information was introduced is shocking. It highlights a significant gap in AI’s understanding, showing they may not yet be reliable for tasks requiring high accuracy. This is crucial for real-world applications.
The realization that sophisticated AI models still fail at seemingly simple tasks emphasizes the need for better structures. As AI progresses, ensuring reliability in various scenarios, especially critical ones, becomes vital.
Challenges in AI’s Future Development
AI’s reasoning shortcomings highlight the challenge of developing smarter systems. Scaling data and models might not be the solution. Apple’s research suggests that true logical reasoning might need new approaches beyond just more data and computing power.
The path to developing AI with real-world applicability is fraught with challenges. Apple’s bold stance provides a roadmap for addressing deficiencies, suggesting that pattern recognition and true reasoning are distinct hurdles to overcome.
Implications of Apple’s Revelations
Apple’s research could significantly impact AI development strategies. If pattern matching is all AI achieves, then it stands at a crossroads. The need for models that understand and reason logically is more pressing than ever.
AI’s future might hinge on bridging the gap between pattern recognition and reasoning. Apple’s insights may spark a shift in how AI is trained and evaluated, pushing for advancements in reasoning capabilities.
Time for a Paradigm Shift?
The findings call for a re-examination of current AI systems. If changing names impacts performance, we need to rethink our approach. AI must evolve to handle complexity without falter, ensuring dependable outputs in varying contexts.
In summary, Apple’s research is a wake-up call for the AI community. It challenges the status quo, calling for a deeper understanding of AI’s capabilities. As the world inches closer to AI integration, ensuring accurate and reliable systems is paramount.