DataGemma AI Open Models Connecting LLMs to Googles Data Commons
3 min read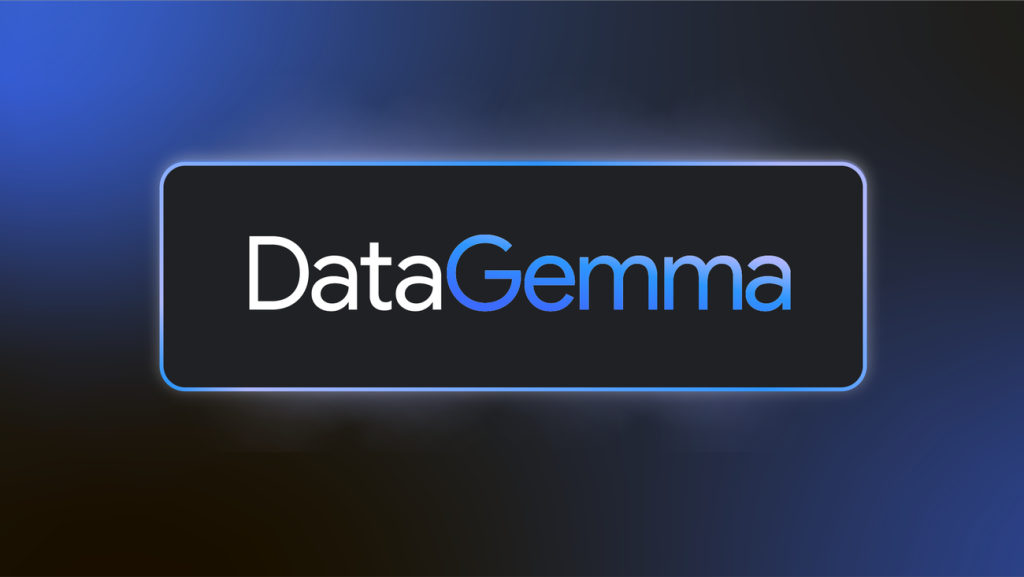
The rise of generative AI has brought remarkable advancements, but it’s not without challenges. One significant issue is the phenomenon known as “hallucination,” where AI confidently presents false information.
DataGemma aims to mitigate this by grounding large language models (LLMs) in real-world data from Google’s Data Commons. This development promises to enhance the factual accuracy of AI responses, making them more reliable and informative.
Data Commons: A Vast Repository of Trustworthy Data
Data Commons is a publicly available knowledge graph that houses over 240 billion rich data points across numerous statistical variables. This vast resource pulls data from trusted sources like the United Nations, World Health Organization, Centers for Disease Control and Prevention, and Census Bureaus.
This unified database provides a wealth of reliable, public information on various topics ranging from health to economics. Users can interact with the data in their own words using an AI-powered natural language interface, exploring questions like the correlation between income and diabetes in U.S. counties.
How Data Commons Tackles Hallucination
By integrating Data Commons within Gemma, DataGemma models aim to ground generative AI experiences in factual data. These lightweight, state-of-the-art open models, built from the same research as the Gemini models, are now available to researchers and developers.
DataGemma employs two distinct approaches to enhance LLM factuality: Retrieval-Interleaved Generation (RIG) and Retrieval-Augmented Generation (RAG). RIG queries trusted sources and fact-checks against Data Commons, while RAG incorporates relevant context beyond training data, enriching the model’s responses.
DataGemma’s Unique Methodologies
RIG enhances Gemma 2 by retrieving statistical data from Data Commons when generating responses. This proactive approach minimizes the risk of hallucinations by ensuring the information is accurate and reliable.
In contrast, RAG uses a long context window to absorb and include more contextual data before generating responses. This results in more comprehensive and informative outputs, reducing the AI’s chances of presenting incorrect information.
For example, when asked about the increase in renewable energy use, both RIG and RAG methodologies ensure that the response is backed by authoritative data from Data Commons.
Promising Results of DataGemma
Initial findings with RIG and RAG show promising enhancements in the accuracy of AI handling numerical facts. This improvement suggests fewer hallucinations in various use cases, benefiting researchers, decision-makers, and curious minds alike.
Ongoing research aims to refine these methodologies, scale up the work, and integrate the enhanced functionality into both Gemma and Gemini models through a phased, limited-access approach.
Future Directions and Aspirations
DataGemma’s open model seeks to facilitate broader adoption of these techniques, grounding LLMs in factual data. The goal is to make AI more reliable and trustworthy, ensuring it serves as an indispensable tool for everyone.
Researchers and developers can get started with DataGemma through quickstart notebooks for both the RIG and RAG approaches, enabling them to explore the potential of these advanced methodologies.
By continuously refining these methods, DataGemma aims to foster informed decisions and a deeper understanding of the world through accurate information grounded in real-world data.
Getting Started with DataGemma
Researchers eager to explore DataGemma’s capabilities can access quickstart notebooks for RIG and RAG, providing a hands-on approach to understanding these advanced methodologies.
DataGemma stands at the forefront of addressing AI hallucination by anchoring LLMs to real-world data from Google’s Data Commons. This innovative approach promises to enhance the accuracy and reliability of AI-generated responses.
As research progresses, DataGemma is set to become an essential tool for providing accurate, data-driven insights, ultimately empowering users with truthful information and fostering informed decisions.