Exploring the Distinct Realms of AI and ML
3 min read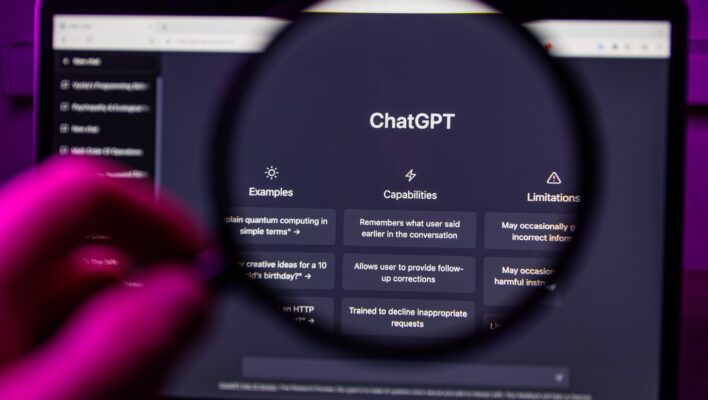
The terms Artificial Intelligence (AI) and Machine Learning (ML) are often tossed around as though they are synonyms, yet their scopes and implications in technology diverge significantly. As we delve deeper into their concepts, it becomes clear that while they share connections, their applications and functionalities are distinct.
Understanding the nuances between AI and ML is not just academic—it’s crucial for anyone interacting with modern technology. This exploration into their definitions, relationships, and individual applications sheds light on their unique roles in the advancement of tech-driven solutions.
Defining Terms: Artificial Intelligence and Machine Learning
Artificial Intelligence (AI) and Machine Learning (ML) are often used interchangeably, but they have distinct characteristics. AI is a broad field encompassing the development of computer systems to perform tasks requiring human intelligence. These tasks include learning, reasoning, and problem-solving. ML is a subset of AI focused on giving computers the ability to learn from and interpret data without being explicitly programmed.
The Relationship Between AI and ML
It’s crucial to understand that all machine learning is artificial intelligence, but not all artificial intelligence is machine learning. While ML is an application of AI, it specifically deals with algorithms and statistical models that allow systems to improve their performance based on the data they process.
This means ML systems can adapt to new scenarios without human intervention, making them exceptionally efficient at tasks such as predictive analysis and pattern recognition.
Applications of AI Without ML
Not all AI systems rely on machine learning. Some use rules or logic-based algorithms to perform specific tasks, known as rule-based systems. These systems operate under pre-defined rules and don’t adapt or learn from new data.
Examples of rule-based AI include chatbots programmed with specific responses or automation software that follows complex rules. These systems are often employed in controlled environments where variables don’t change frequently.
Although less flexible, these AI applications are crucial for tasks requiring high reliability and consistency, such as in regulatory compliance or where changes in data can’t be easily predicted.
How ML Advances AI
Machine learning enhances AI capabilities by enabling systems to learn from data, improve their algorithms, and make more accurate predictions over time. This learning process is fundamental for applications like image recognition, where precision increases with the amount of processed data.
One example is facial recognition technology, which has evolved significantly thanks to machine learning. As the system ingests more images, its ability to distinguish between different faces improves.
Challenges in Distinguishing AI from ML
The overlap between AI and ML can sometimes create confusion. It’s often hard to draw a clear line where AI ends and ML begins because many AI advancements today involve some form of machine learning.
For instance, autonomous driving technology uses both AI for decision-making and ML for improving its understanding of the driving environment.
In such cases, the integration of both technologies is crucial for developing sophisticated systems that can handle complex environments.
The Future of AI and Its Subfields
The future of AI is likely to witness increased blending of various subfields, including ML, making it more challenging to differentiate them. As technology advances, AI and ML will continue to integrate, potentially leading to new subsets within AI itself.
This integration signifies not just technological advancement but also a shift in how we conceptualize what machines are capable of achieving. It raises important questions about the roles of AI and ML in future developments.
In conclusion, the realms of Artificial Intelligence (AI) and Machine Learning (ML) are intricately linked yet distinct, each with its own unique processes and applications. While ML operates as a subset within the broader AI landscape, enhancing predictive and analytical capabilities, AI encompasses a wider array of technologies including rule-based systems which do not require learning. As technology progresses, understanding these distinctions becomes crucial for leveraging their potential in innovative and responsible ways.