Innovative Robot Training: How iPhone Scans are Revolutionizing Home Automation
5 min read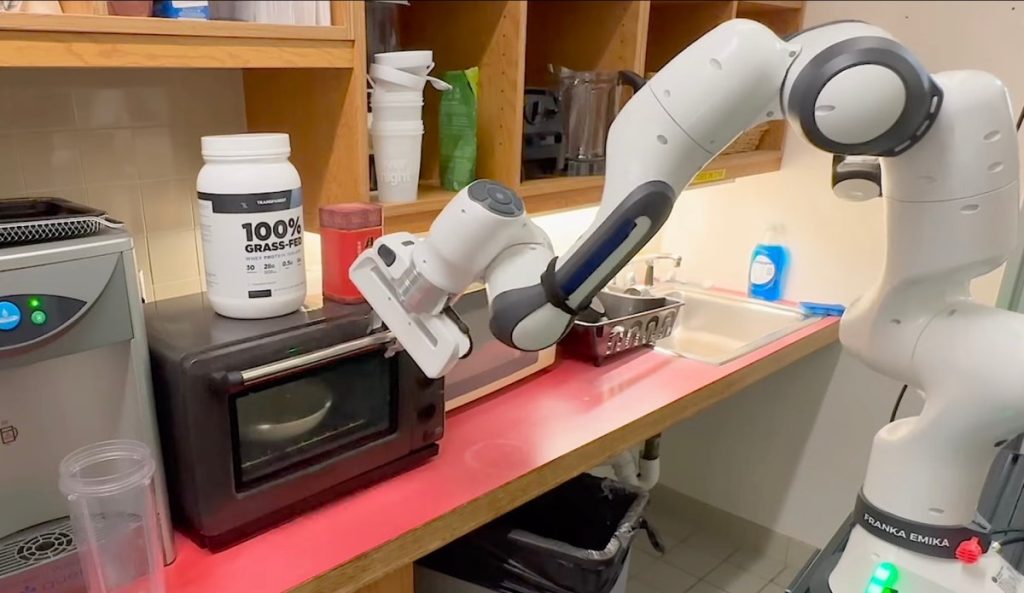
In the ever-changing landscape of home automation, researchers are unveiling groundbreaking methods to enhance robot training. At MIT’s CSAIL, a new approach is being presented, harnessing the power of iPhone scans to create realistic simulations of home environments. This technology shift is a game-changer.
Through these simulations, robots can master tasks in a risk-free virtual world. This advancement not only enables safer learning but also promises a future where robots adapt seamlessly to our dynamic, everyday spaces.
Training Home Robots: The Simulation Shift
The task of training home robots has long been challenging due to the unpredictable environments they must navigate. MIT’s CSAIL has pioneered a new method using iPhone scans to create simulations of real home environments. The simulation approach allows robots to safely and efficiently learn tasks. By practicing in a virtual world, robots can make countless mistakes without real-world consequences.
Previously, the variability in home layouts and the presence of humans and pets presented obstacles. No two homes are the same, and even small changes can throw off a robot. However, with the new simulation technology, robots can adapt better to different settings. They get used to unexpected changes like moved furniture or objects left around.
Why Simulation is Crucial
Simulation has become vital in robot training. It lets robots practice tasks repeatedly in a controlled environment. For example, a robot learning to load a dishwasher might virtually break countless dishes. This trial and error method helps perfect its accuracy and efficiency. As researcher Pulkit Agrawal points out, “It might have broken a thousand dishes, but it doesn’t matter because everything was in the virtual world.”
The consequences of failure in real life would be too costly and impractical. By using simulations based on iPhone scans, robots gain a robust understanding of various home environments. This method smoothens the pathway for future developments in home robotics, making it more feasible for everyday use. More critical, it helps the robots become more adaptable and useful over time.
Researchers can now build comprehensive databases of different home environments using these scans. This data collection is essential for improving robot training. The more diverse the environments, the better robots can handle unexpected changes. Therefore, this technology is not just an advancement; it’s a milestone in making home robots practical and reliable.
Adapting to Dynamic Environments
Home environments are dynamic and always changing, which is a tricky aspect for robots to handle. The ability to adapt to these changes is a significant development. With each new scan of a home layout, robots update their data and improve their adaptability.
This flexibility means that robots can perform their tasks efficiently, even when things are moved around. For instance, if a chair is shifted or new objects are introduced, the robot can adjust its actions based on the latest scan. This adaptability is crucial for practical home use.
Technical Process and Methodology
The technical process behind this involves scanning a portion of the home using an iPhone. The scan is then uploaded to the simulation software, creating a virtual replica of the environment. Scientists can use these replicas to train robots repeatedly in various tasks without any real-world risks.
The simulations are not just static images but dynamic environments where robots can interact with objects. This interaction helps improve the robot’s decision-making skills and task execution. Robots learn not only the layout but also how to react to changes. This makes the learning process more realistic and effective.
Researchers aim to make these simulations as detailed and lifelike as possible. The more realistic the simulation, the better the training for the robot. This method bridges the gap between theoretical training and practical application, making robots more prepared for real-world scenarios.
The Importance of Data Collection
Collecting varied data is essential for the success of this training method. Each new environment added to the simulation database increases the robot’s ability to generalize its learning. More data means better performance and adaptability.
The data also helps identify areas where the robot might struggle. By analyzing the robot’s performance in different scenarios, researchers can tweak the training process. This continuous improvement loop is vital for developing reliable home robots.
Benefits for the Future of Home Robots
The implications of this technology are vast. By making it easier to train robots, it speeds up the development process. This could lead to more advanced and capable home robots in a shorter time frame.
As the technology progresses, we can expect home robots to become more common. They will be able to perform a wider range of tasks, making everyday life more convenient. The potential for this technology to transform home living is immense.
The successful training of robots using this method could also inspire further innovation in the field. It sets a new standard for how robots can be trained and deployed in various environments outside the home, such as in healthcare or service industries.
Challenges and Future Directions
Despite its promise, this technology has its challenges. One of the main issues is ensuring the accuracy and detail of the iPhone scans. If the scans lack precision, the simulation might not be effective.
Researchers are working on improving scanning technology to overcome these hurdles. Higher accuracy in scans means better training for the robots. This continuous improvement is crucial for the long-term success of home robotics.
In summary, MIT’s approach of using iPhone scans for training home robots showcases an innovative leap forward in the realm of home automation. This method not only provides a risk-free environment for robots to learn and adapt but also holds massive potential for future developments. With ongoing improvements, this technology could revolutionize how we interact with robots in our daily lives.
Although challenges remain, the progress made thus far is promising. The ability to efficiently train robots for dynamic home environments means a more practical and user-friendly future for home robotics. The advancements in this field could lead to significant enhancements in convenience and efficiency within our homes.