Inside the Secretive World of OpenAI’s Revolutionary Model
3 min read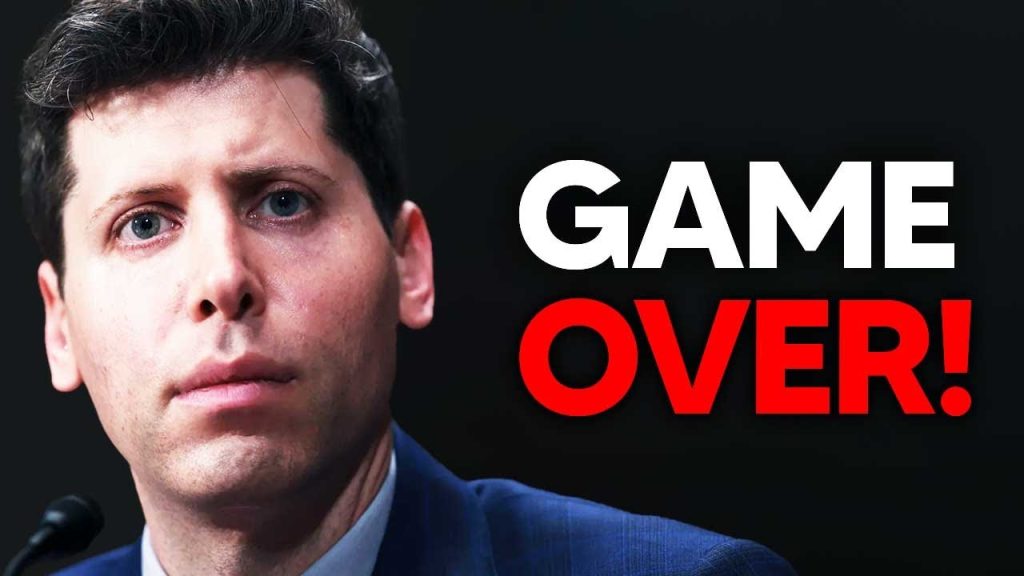
OpenAI has captivated the world with its groundbreaking AI model, the 01 series. But what makes it so advanced?
The model is cloaked in secrecy, raising curiosity around how it functions and its path towards Artificial General Intelligence (AGI).
Breaking Down the Fundamentals
At the heart of the 01 series is reinforcement learning, akin to training a dog with treats for desired actions. The AI gets digital rewards for completing tasks correctly.
Reinforcement learning is crucial for equipping the AI with reasoning skills, enabling it to tackle complex problems through trial and error. The basics of the model involve policy initialization, reward design, search capabilities, and continuous learning.
The Role of Policy Initialization
Imagine preparing to play chess by first understanding the rules. Policy initialization shapes the foundational skills of the AI before tackling tougher problems.
OpenAI begins with massive pre-training on text data, akin to reading vast swathes of the internet. This gives the AI a broad knowledge base.
The process progresses with fine-tuning using prompt engineering and supervised learning, teaching the AI to reason and solve specified problems.
Reward Design: Crafting Motivation
Reward systems guide the AI’s learning journey, providing motivation to pursue correct solutions.
Outcome reward modeling judges the final product, while process reward modeling assesses each step, rewarding correct ones and penalizing errors.
Refining solutions on a granular level facilitates iterative improvement, crucial for complex reasoning tasks.
Search: The AI’s Problem-Solving Core
Search allows the AI to explore various possibilities, akin to brainstorming multiple drafts before finalizing an essay.
Tree search and sequential revisions are strategies OpenAI employs, guiding the AI to review potential choices and refine its solutions. Each step fine-tunes the approach to problem-solving.
Internal and external guidance support the search process, with the AI’s own understanding and external feedback steering its exploration.
AI’s self-evaluation capability lets it double-check its work, ensuring confidence in its conclusions at every stage.
The reward model further enhances search by offering feedback, guiding the AI to better outcomes.
Continuous Learning: Evolving Intelligence
Reinforcement learning enables ongoing improvement, utilizing rewards to tweak the AI’s decision-making process.
Policy gradient methods like PO allow the AI to adjust its strategies, making success more likely over time.
Behavior cloning helps the AI mimic effective solutions, strengthening its repertoire for problem-solving scenarios.
Combining search and learning creates a loop of practice and feedback, essential for superhuman performance.
The AI’s ongoing cycle of learning allows it to evolve, potentially surpassing human problem-solving capabilities.
Are We Nearing Super Intelligence?
Insights from the latest research suggest that super intelligence might not be far off.
Continuous search and learning could enable AI to achieve unprecedented levels of reasoning.
Many believe OpenAI could be paving the way for breakthroughs in AI capabilities.
The Chinese Research Impact
A recent study by Chinese researchers hints at unraveling the secrets behind OpenAI’s model.
This discovery could democratize AI development, letting more players compete with OpenAI.
Implications for the Future of AI
If the insights from China prove accurate, we might witness a surge in advanced AI models globally.
This could level the field and spur innovation, reshaping how AI is developed and utilized.
The unfolding story of OpenAI’s advancements could redefine our approach to AI. Are we ready for what’s next?