Many Safety Evaluations for AI Models Have Significant Limitations
3 min read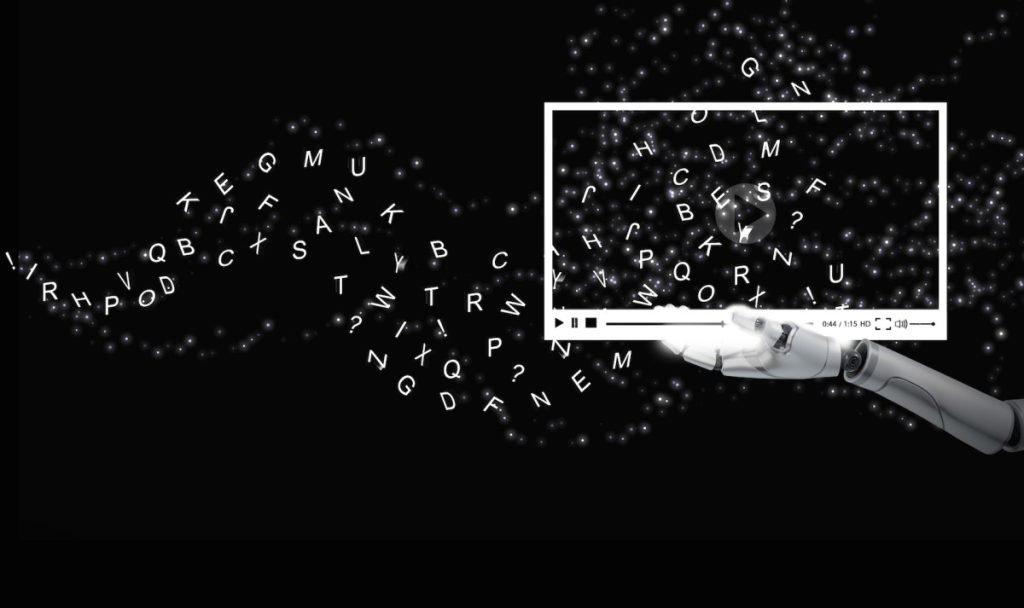
Despite increasing demand for AI safety and accountability, current tests and benchmarks for AI models may fall short, according to a new report.
generative AI models are under increased scrutiny for their tendency to make mistakes and behave unpredictably. Organizations propose new benchmarks to test these models’ safety.
Benchmarks and Red Teaming
The study’s authors first surveyed academic literature to understand the current state of AI model evaluations. They interviewed 16 experts, including employees from tech companies developing generative AI systems. Their findings showed sharp disagreements within the industry on the best methods to evaluate models.
Some tests only examined how models aligned with benchmarks in the lab, not how they would impact real-world users. Others used tests developed for research, not evaluating production models. However, vendors still insisted on using these in production.
Experts noted it’s tough to determine a model’s performance from benchmark results. For instance, a model may perform well on a state bar exam but fail to solve real-world legal challenges. Data contamination is also a significant issue, where benchmark results can overestimate a model’s performance if trained on the same data used for testing.
Benchmarks are often chosen for convenience rather than effectiveness. “Benchmarks risk being manipulated,” said Mahi Hardalupas, researcher at the ALI. Developers might train models on the same data set used for evaluation, similar to seeing the exam paper before the test. Small changes can also cause unpredictable behavior changes and may override safety features.
The ALI study found issues with ‘red-teaming,’ where individuals or groups try to find vulnerabilities in a model. Many companies, including OpenAI and Anthropic, use red-teaming. However, few standards exist for red-teaming, making it hard to assess its effectiveness. Finding people with the necessary skills and expertise is also challenging, and the manual nature of red-teaming makes it costly and laborious.
Possible Solutions
One of the main reasons AI evaluations haven’t improved is the pressure to release models quickly. “A person we spoke with developing foundation models felt there was more pressure to release models quickly, making it harder to take evaluations seriously,” said Jones.
Major AI labs are releasing models faster than society can ensure they are safe and reliable. Evaluating models for safety is considered an ‘intractable’ problem by some experts.
Mahi Hardalupas believes there is a path forward but says it requires more engagement from public-sector bodies. “Regulators and policymakers must clearly articulate what they want from evaluations,” he said. The evaluation community must also be transparent about the current limitations and potential of evaluations.
Hardalupas suggests governments mandate more public participation in developing evaluations. He also recommends supporting an ecosystem of third-party tests, including programs to ensure regular access to required models and data sets. Jones thinks developing ‘context-specific’ evaluations that look at the types of users a model might impact and how attacks could defeat safeguards is necessary.
Behind those recommendations, there’s a call for more investment in the underlying science of evaluations. This is to develop more robust and repeatable evaluations based on understanding how an AI model operates.
However, there may never be a guarantee that a model is entirely safe. According to Hardalupas, ‘safety’ is not a model’s inherent property. It depends on the context in which it is used, who accesses it, and if the safeguards are adequate and robust.
Evaluations can help identify potential risks but cannot guarantee a model is perfectly safe. Many experts agree that evaluations can only indicate a model is unsafe, not prove it is safe.
In summary, while AI evaluations are essential, they currently have significant limitations. These tests can indicate potential risks but can’t guarantee complete safety.
Moving forward, greater involvement from public-sector bodies, better transparency, and context-specific evaluations can help improve the reliability and safety of AI models.