New Tool Developed to Tackle AI ‘Hallucinations’: A Major Breakthrough in AI Technology
4 min read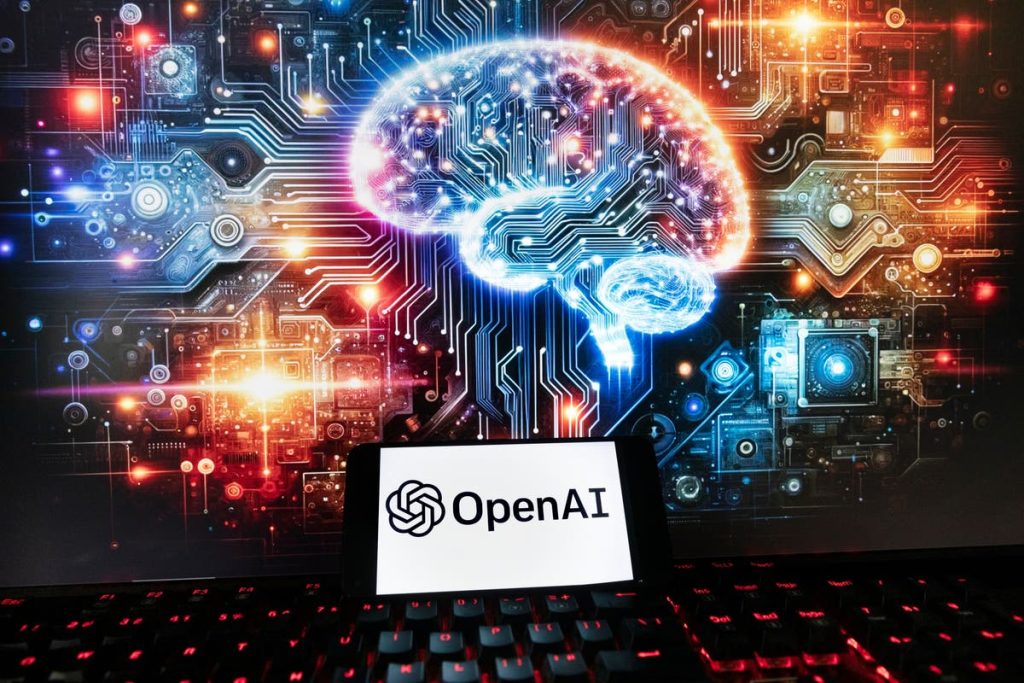
In the ever-evolving field of artificial intelligence, a groundbreaking development has emerged. Scientists have potentially discovered a method to counter the ‘hallucinations’ that frequently challenge popular AI systems, like ChatGPT. These hallucinations are instances when AI generates information that seems real but is entirely fabricated.
This new tool holds the promise of identifying when these large language models (LLMs) are producing unreliable data. It’s a significant leap forward, as LLMs are traditionally designed to generate human-like language without a focus on factual accuracy. Consequently, this innovation could dramatically reduce the instances of misleading outputs and enhance the reliability of AI systems.
Scientists Discover New Tool to Tackle AI ‘Hallucinations’
Scientists may have developed a method to tackle one of the biggest issues plaguing popular artificial intelligence (AI) systems: ‘hallucinations’. This problem arises in large language models (LLMs) like ChatGPT, where AI sometimes produces information that appears legitimate but is actually fabricated.
The newly developed tool aims to identify when LLMs are ‘hallucinating’ or generating information without a factual basis. It’s a major advancement because, currently, LLMs are designed to generate human-like language, not necessarily accurate information. This new method could significantly reduce the number of these misleading outputs.
When LLMs don’t have enough knowledge to answer a question, they often create convincing but inaccurate responses. The innovative solution involves using another LLM to cross-check the initial output, with a third model evaluating this cross-check. This multi-step verification approach helps distinguish reliable information from ‘hallucinations’.
How the System Works
The unique system doesn’t just check words; it focuses on meanings. Researchers feed the questionable output from one LLM into another, which then determines if the statements imply the same idea or not. This process essentially looks for paraphrases to measure the credibility of the original output.
Further research showed that the results from a third LLM, which evaluates this paraphrasing check, are quite similar to human evaluations. This technique is like ‘fighting fire with fire,’ according to one researcher, using LLMs to self-regulate their own errors.
The tool’s reliability is a significant step forward. By ensuring that AI-generated information is trustworthy, this system could expand the range of applications where LLMs can be used, from customer service to medical advice.
Potential Implications and Risks
While this system offers promising advancements, scientists also caution about its potential risks. Utilizing multiple LLMs could inadvertently amplify errors if not managed properly.
According to Karin Verspoor from the University of Melbourne, there’s a concern that layering multiple systems prone to errors might not fully resolve the issue. Instead, it could introduce new complexities.
As the research evolves, it’s crucial to continually evaluate whether this approach genuinely controls LLM outputs or creates more unpredictable results. Balancing these factors will be key to its successful implementation.
Importance for Broader AI Applications
Improving the reliability of LLMs has far-reaching implications. These models can be used in various fields, including healthcare, finance, and education. Ensuring accuracy is vital for their effectiveness in these areas.
With this new system, AI models could become more dependable, making them suitable for tasks requiring high levels of trust. For instance, providing accurate medical advice or legal information.
As sectors increasingly adopt AI technologies, having a reliable method to eliminate ‘hallucinations’ could foster broader acceptance and integration of LLMs.
Research and Publication
The research behind this new tool is detailed in a paper titled ‘Detecting hallucinations in large language models using semantic entropy’, published in Nature.
This paper outlines how the new method works and its potential benefits and dangers. As the field of AI continues to grow, such research is essential for developing safer and more effective AI systems.
Continued exploration and refinement of these techniques will be necessary to stay ahead of the challenges posed by advanced AI technologies.
Expert Opinions
Experts have weighed in on this development, noting both its potential and its limitations. The consensus is that while the tool is promising, it’s not a foolproof solution.
The primary concern remains whether this method can genuinely prevent ‘hallucinations’ or if it merely offers a temporary fix. Ongoing research will be required to address these questions satisfactorily.
Nevertheless, the innovation represents a step forward in making AI more reliable and trustworthy. This is particularly important as AI becomes more integrated into everyday life.
Future Directions
Looking ahead, the research community is focused on refining this tool to ensure its effectiveness. Addressing both its strengths and potential pitfalls will be crucial for its success.
Future studies will likely explore additional layers of verification and new techniques to further minimize the chances of AI-generated ‘hallucinations’.
The ultimate goal is to create AI systems that are both innovative and safe for widespread use. Ensuring accuracy and reliability will be at the forefront of these efforts.
Concluding Thoughts
The development of this tool marks an important step in the ongoing effort to improve the reliability of AI systems. By identifying and reducing ‘hallucinations’, this method holds promise for expanding the safe use of AI technologies across various fields.
The development of this tool marks an important step in improving AI’s reliability. By identifying and reducing ‘hallucinations’, the method expands the safe use of AI technologies across various fields. Continued research will be crucial for making these systems more trustworthy.
Ultimately, this innovation not only enhances the practical applications of AI but also builds greater public confidence in these technologies. Future advancements will likely refine and enhance this tool, making AI an even more integral part of our daily lives.