Nvidia’s Llama 3.1 Open-Source AI Outshines the Giants
3 min read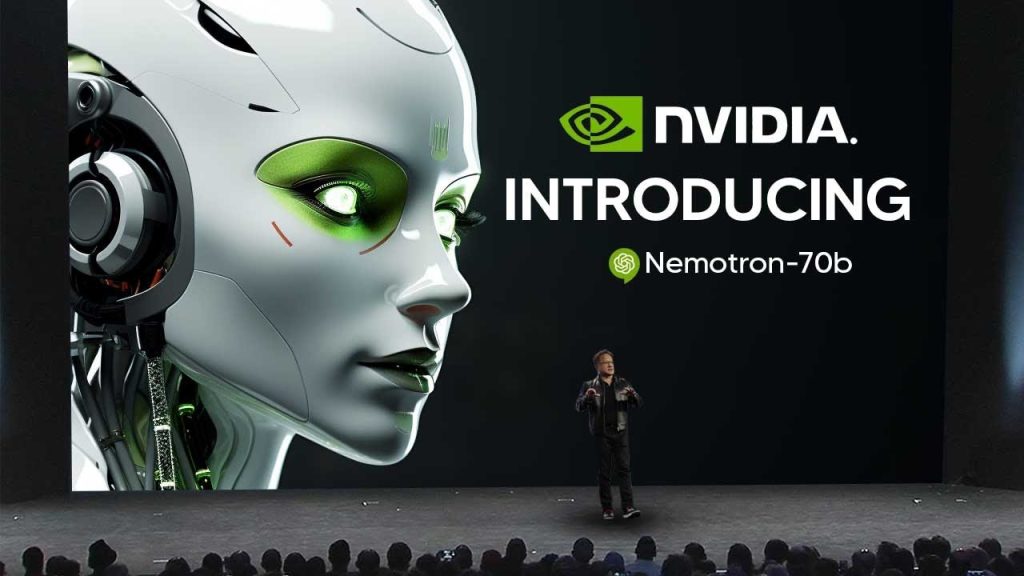
Nvidia’s latest AI model, Llama 3.1 Neaton 70B, has astounded the tech world. This open-source model, with its astounding capabilities, has outperformed many closed-source counterparts.
By utilizing innovative reward modeling and reinforcement learning, Nvidia has set a new standard for AI performance. Let’s dive into how this remarkable achievement unfolded.
Nvidia’s Stunning Breakthrough
Nvidia’s release of the Llama 3.1 Neaton 70B model has turned heads, surprising many by surpassing every closed-source model out there. It’s another reminder that open source continues to push the boundaries of what AI can achieve, even when rivaled by closed-source efforts.
Interestingly, Nvidia used the Llama 3.1 as a base and applied post-training with reinforcement learning. This approach allowed them to create a model that outperformed state-of-the-art models like GPT-40. It’s a testament to the potential of novel training methods.
The Reward Model Innovation
A key to this success lies in the innovative approach to reward modeling. Nvidia explored two main styles: the Bradley Terry style and the regression style. These methods aim to deliver more accurate AI responses by assigning reward scores.
The challenge was balancing these models, trained on different data types. Nvidia tackled this by introducing a dataset called Help Steer 2, which includes preference and numeric rankings, bridging the gap between the two methods.
Achieving Top Benchmarks
Combining these methodologies resulted in Llama 3.1 Neaton 70B achieving top scores on several benchmarks, including the Arena Hard Auto.
Arena Hard Auto is an evaluation tool with 500 challenging queries, showcasing the model’s prowess without style control aspects, which often alter user perception of helpfulness.
The model edged out renowned counterparts like GPT-4 Turbo, showcasing its ability to stand out, even amongst close rivals. This demonstrates Nvidia’s leap in AI innovation.
Testing Real-World Scenarios
In real-world scenarios, the model’s ability to reason and decipher questions, even with irrelevant information, was put to the test. It showed significant capability, albeit with some hiccups.
In one instance, the model initially stumbled over a question with misleading information but, upon reevaluation, managed to provide an accurate response. Such instances highlight the power of clever prompt engineering.
Improvements and Discoveries
The model’s improved reasoning was evident in tests involving deceptive queries about daily objects. Notably, it handled a question on kiwi counting adeptly, showcasing its keen understanding when compared to other models that struggled.
This sophistication in tackling reasoning questions stems from advanced reward modeling, which fine-tuned the model’s analytical capabilities to match real-world expectations.
The success reaffirms the importance of precise prompt engineering, emphasizing the model’s innate smartness that can be drawn out with the right guidance.
Curious Capabilities
One of the model’s quirks lies in its ability to correctly answer whimsical prompts, such as counting letters in ‘strawberry,’ a task other models failed.
This ability, while seemingly trivial, underscores the model’s strength in detailed analysis and problem-solving, bridging the gap between novelty and practical utility.
Nvidia’s strategy in reward modeling could set a benchmark for future models, pushing boundaries previously imagined.
The Future with Open Source Models
Open source models like Llama 3.1 continue to challenge closed-source counterparts, often leapfrogging ahead with breakthrough innovations.
The race doesn’t stop here. With continuous advancements, there remains an expectancy of even more sophisticated models on the horizon.
Nvidia’s recent success suggests a future where open source consistently captivates the lead in AI development.
Nvidia’s Llama 3.1 Neaton 70B has highlighted the prowess of open-source in AI.
As these models continue to evolve, we’re likely to witness even greater feats in the AI landscape.