OpenAI Faces Challenges in AI Advancement A Look into the Future of AI Models
4 min read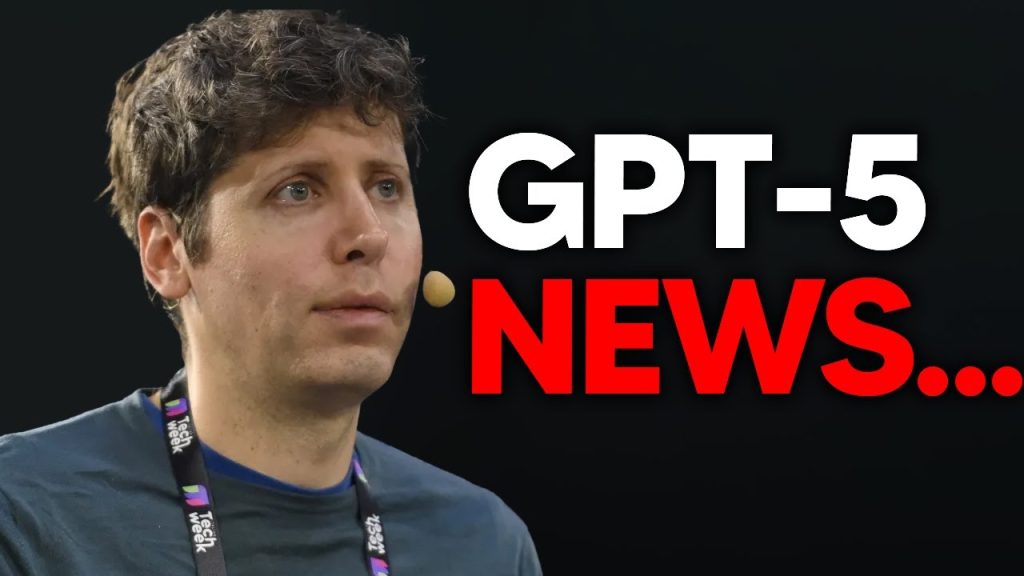
OpenAI is encountering hurdles on its path to Artificial General Intelligence (AGI). The company is rethinking its strategy as improvements in its AI models slow down.
An article from The Information raises questions about AI’s ability to learn and whether its intelligence can keep growing. Some within the AI community worry if these models are hitting their limits.
The Orion Model: A Mixed Bag
OpenAI’s upcoming AI model, Orion, is not proving to be as revolutionary as anticipated. While it excels in language tasks, it’s not significantly better at coding than its predecessors, raising concerns about its cost-effectiveness.
Orion was supposed to be a game-changer. Yet, some within OpenAI admit it isn’t consistently outperforming past models. This inconsistency, especially in coding tasks, is raising questions about the model’s efficiency and whether it justifies its higher running costs.
Internal Models and Coding Capabilities
OpenAI has been developing an internal model for handling software engineering tasks, which has shown promise within the company.
These internal tools are designed to handle complex coding tasks that typically take humans hours to complete. But it’s still unclear if these tools will ever be available publicly, indicating a possible strategic shift within OpenAI.
OpenAI’s internal coding model highlights a divide between what the company is developing for internal use and what’s available for public consumption. Despite its capability, the Orion project struggles to deliver similar results, prompting questions about the eventual public accessibility of these advanced tools.
Scaling Laws and Paradigm Shift
The article highlights that Orion challenges the longstanding belief in the AI field: scaling laws.
For years, it was believed that AI models would continue to improve if given more data and computing power. Yet, Orion’s performance suggests a possible slowdown, sparking debates about future AI advancements.
This raises big questions. If AI models hit a plateau, what does this mean for the industry’s growth relying on scaling laws? As the paradigm shifts, companies might need to rethink their strategies, potentially affecting investment in AI infrastructure.
While many in AI have trusted scaling laws to drive progress, Orion’s development invites doubt. This shift in perception could influence how AI companies plan their research and resource allocation in future projects.
Test-Time Compute: A New Hope?
OpenAI is focusing on improving models after their initial training. This “test-time compute” strategy aims to yield better-performing AI models.
While traditional scaling relied on more data and time, test-time compute diverges. It focuses on enhancing AI performance through different methods, potentially offering better results for lower costs.
This new method highlights a strategic pivot for OpenAI. Instead of relying solely on more data, the company is investing in smarter training methods that might provide better outcomes.
Minor Improvements Still Matter
Even minor improvements in AI models can unlock new use cases. Orion, though not dramatically superior, could still provide valuable advancements.
Every new AI iteration seeks to resolve previous issues. Even if progress seems small, it often translates to real-world applications. Orion might surprise despite its seemingly modest upgrades.
The seemingly incremental changes from Orion can pave the way for innovative uses. For AI enthusiasts, even slight advancements can significantly impact various industries, proving the continued importance of AI developments.
Challenges of Data Quality
The data wall is a real concern for AI development. High-quality data is getting scarce, impacting AI training.
Finding good data has always been tough. Most online information isn’t ideal for training models, complicating efforts to enhance AI capabilities.
OpenAI is working on improving data quality. By creating synthetic data, they aim to refine their training process, even if it presents new challenges.
Synthetic Data: A Double-Edged Sword
Orion’s development has benefited from using synthetic data, created by previous AI models.
Though this strategy boosts training, it risks making Orion too similar to its predecessors, which might limit its uniqueness.
Despite these challenges, synthetic data remains a promising avenue for AI training. Balancing innovation with stability is key to future breakthroughs.
Economic Realities and Future Prospects
AI development costs are rising. While models advance scientific research, their costs hinder widespread adoption.
As models become pricier, their accessibility is questioned. OpenAI’s current models have limited customer bases due to their cost, but future improvements may lower expenses.
Despite upfront costs, AI’s potential benefits could balance the scales over time. As pricing becomes more competitive, broader user adoption is likely.
A Competitive AI Landscape
The AI race is fierce, with companies like Google and Meta also pushing boundaries.
OpenAI isn’t alone in facing challenges. Competitors are exploring similar problems, signaling a dynamic future for AI development.
The journey towards smarter AI models is full of challenges and opportunities. While OpenAI navigates uncertainties, its focus on innovative strategies might set the stage for future breakthroughs.