OpenAI’s New Model CriticGPT Aims to Spot AI Mistakes
3 min read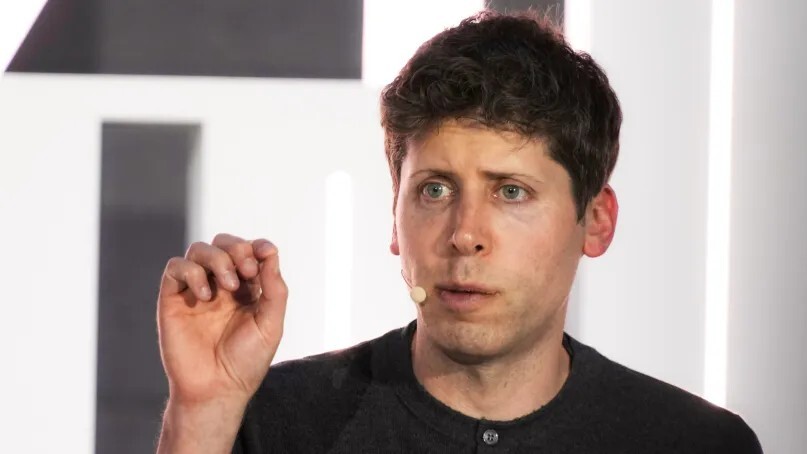
In the quest to improve the accuracy of AI models, OpenAI has devised an innovative solution: CriticGPT. CriticGPT is a new model designed specifically to catch mistakes made by other AI models. It’s an interesting approach because CriticGPT is powered by the same technology it aims to scrutinize, GPT-4.
AI models often produce erroneous responses, and typically, humans step in to identify and correct these errors. However, as AI models get more advanced, this task becomes increasingly difficult. CriticGPT has shown promising results in evaluating coding tasks, indicating a significant leap forward in AI accuracy and reliability.
OpenAI’s Approach to Spotting AI Mistakes
OpenAI introduced CriticGPT, a model designed to identify flaws in AI-generated responses. This innovative model is powered by GPT-4. CriticGPT functions as an expert lie detector, trained by human researchers who fed it false information and guided it to respond with detailed critiques. Initially, OpenAI is utilizing CriticGPT to evaluate GPT-4’s coding abilities, where answers are straightforward and easier to judge.
Performance Comparison: AI vs. Humans
While humans are still involved in the assessment process, CriticGPT has demonstrated a higher efficiency in identifying errors. According to tests, CriticGPT detected 85% of coding bugs, whereas trained humans identified only 25%. The combined effort of humans and CriticGPT showed a 60% improvement over human assessment alone. This synergy highlights the potential of AI-assisted human evaluations in enhancing accuracy.
Alternative Approaches to AI Accuracy
Researchers from the University of Oxford have also developed an algorithm that identifies AI-generated hallucinations 79% of the time, which is about 10% more effective than current methods. However, this approach consumes ten times more energy than a typical chatbot interaction, indicating a trade-off between accuracy and energy efficiency.
The Role of Humans in AI Training
Despite advancements in AI, human involvement remains crucial. Human testers help refine AI models by generating multiple responses to the same question and selecting the most relevant and accurate one. This process, however, is becoming more challenging as language models (LLMs) evolve. Human assessments can introduce mistakes and biases, emphasizing the need for improved AI tools like CriticGPT to assist in this task.
CriticGPT’s Future Potential
The success of CriticGPT in evaluating coding abilities opens up possibilities for its application in other domains. As the model continues to evolve, it could become a valuable tool for assessing open-ended and subjective responses, thereby further enhancing the overall accuracy of AI models. The collaborative approach of combining human expertise with AI detection capabilities shows promise for future AI development.
Challenges and Considerations
While AI tools like CriticGPT and the Oxford algorithm offer significant improvements in accuracy, they are not without challenges. The increased energy consumption and the potential for introducing new biases are important factors to consider. Therefore, ongoing research and development are essential to address these issues and optimize the effectiveness of AI detection methods. In conclusion, the integration of AI-assisted human evaluations marks a significant step towards enhancing the accuracy and reliability of AI models, paving the way for more dependable AI applications in various fields.
OpenAI’s development of CriticGPT ushers in a new era of collaboration between humans and AI to make models more accurate. The promising results from CriticGPT highlight its potential to transform various domains beyond coding. Though there are challenges, ongoing research and innovation are essential for optimizing AI detection methods.
By combining human intelligence with AI’s efficiency, we can enhance the reliability and accuracy of AI systems significantly. This partnership marks a significant advancement in AI technology, paving the way for more dependable applications in the future. The integration of these advancements will likely drive further developments in AI, benefiting multiple fields.