Revolutionizing AI Training with Minimal Energy Consumption
3 min read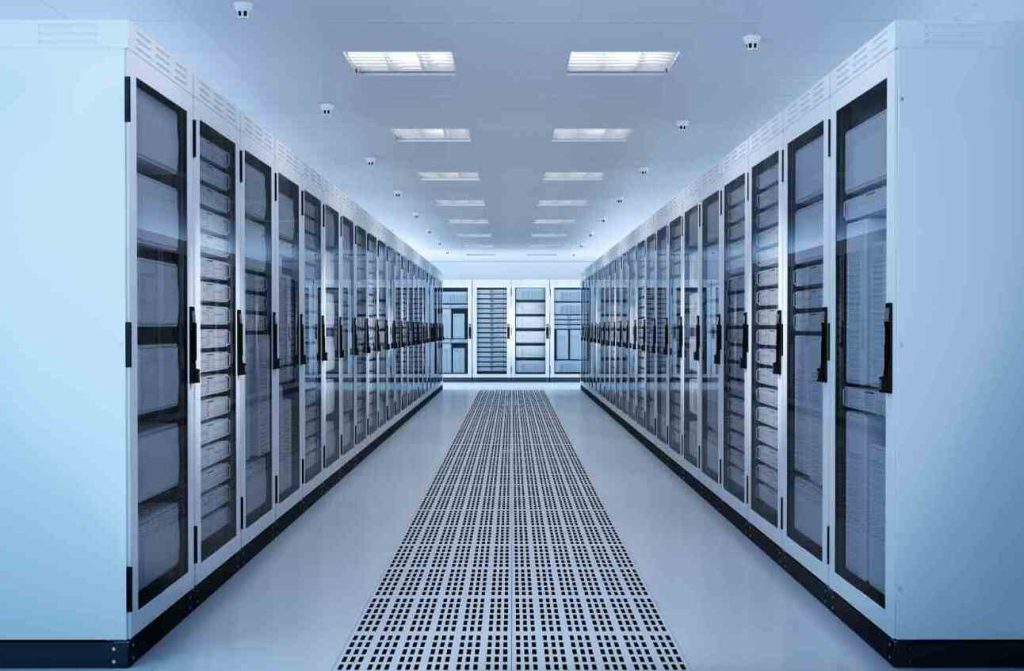
Researchers at UC Santa Cruz have made a groundbreaking discovery. They’ve developed a new method to train AI models using only the energy required to power a lightbulb. This innovative approach could dramatically reshape the AI industry into a more sustainable and efficient field.
Typically, training AI models demands vast computational power, often at a high energy cost. However, this new technique breaks through those constraints, offering a glimpse into a future where AI development is not only powerful but also energy-efficient.
A New Way to Train AI Models
Researchers at UC Santa Cruz have discovered a new method to train AI models using minimal energy. Typically, training AI models require powerful computers that consume a lot of power. However, the new technique uses only the amount of energy needed to power a lightbulb. This breakthrough could transform the AI industry, making it more sustainable and efficient.
Energy Consumption in AI
By 2027, the AI industry is predicted to consume as much energy annually as the entire Netherlands. Current AI models rely on an energy-intensive process known as matrix multiplication (MatMul). This process involves multiplying numbers across several network layers, which can be quite complex and power-hungry.
The Ternary Approach
The researchers have found success by converting numbers into ternary values instead of binary. Ternary values include -1, 0, and 1, making calculations simpler for computers. This approach reduces the complexity of matrix multiplication, significantly decreasing energy consumption.
Impressive Results
The results of this new method are promising. Researchers were able to run a model similar to Llama-2 on a billion-parameter scale using just 13 watts of power, equivalent to an LED light bulb. Remarkably, this was achieved without any significant loss in performance.
Scaling Up
Although the method currently works for billion-parameter models, it is not yet compatible with more extensive trillion-parameter models. However, researchers are optimistic about scaling up this approach and adapting it to existing AI hardware.
Environmental Impact
Addressing the environmental impact of AI is becoming increasingly important. Companies are exploring various solutions, such as atomic fusion and geothermal energy, though these methods may not be viable in the short term. The ternary approach offers a more immediate and practical solution, potentially reducing the carbon footprint of AI training.
Challenges and Future Prospects
One challenge of this new method is its current limitation to billion-parameter models. As AI companies develop more complex models, the need for energy-efficient solutions will only grow. Researchers are confident that with further development, this technique can be scaled up and applied to larger models.
AI Industry Adaptation
Several tech companies are already showing interest in this new method. Although it is still in the early stages, the potential for significant energy savings makes it an attractive option for AI developers. Companies can integrate this approach into their existing hardware, making the transition smoother and more cost-effective.
Broader Implications
The implications of this research extend beyond just energy savings. If successful, it could lead to more sustainable AI practices globally. This would be a significant step forward in the quest for environmentally friendly technology solutions, benefiting both the industry and the planet.
The innovative training method discovered by UC Santa Cruz researchers presents a promising future for AI. This breakthrough in energy-efficient computing could reshape the industry, making AI development more sustainable and accessible.
By converting numbers to ternary values, this novel approach drastically reduces energy consumption without compromising performance. Though challenges remain, the potential benefits make it an exciting advancement.
Looking forward, as researchers refine and scale this technique, the AI industry stands on the brink of a transformative shift toward greener and more efficient practices.