Scientists Warn About the Risks of AI Model Collapse
4 min read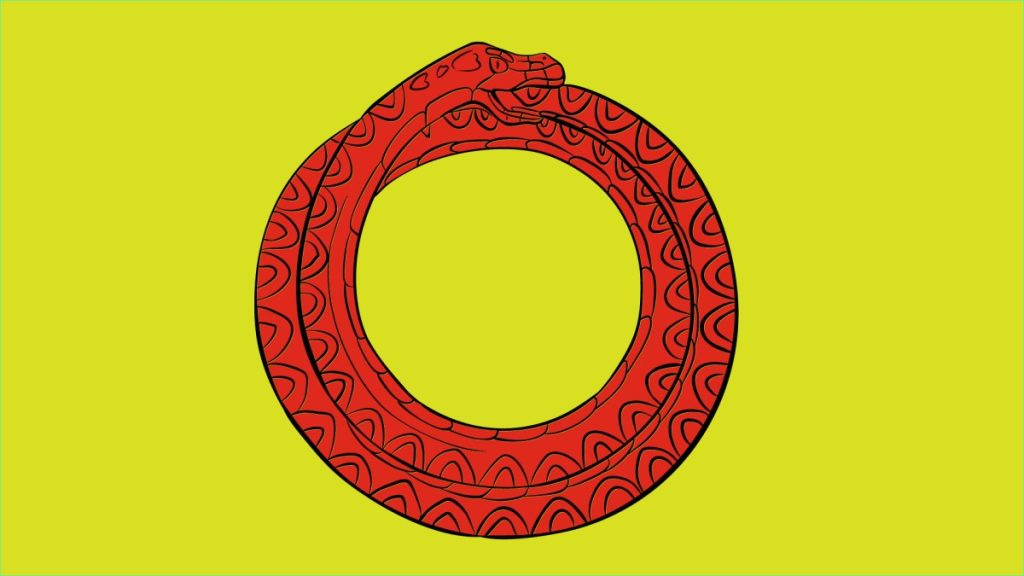
Researchers have raised alarms about a new risk in AI development: model collapse. This phenomenon could lead AI systems to become increasingly unreliable over time, threatening their effectiveness.
Model collapse occurs when AI models learn from data created by other AI, reinforcing inaccuracies and biases. This issue is increasingly concerning as AI-generated content becomes more common on the web.
Understanding Model Collapse
In recent research led by Ilia Shumailov at Oxford, the term ‘model collapse‘ was introduced to describe a worrying trend in AI development. Over time, AI models that learn from data produced by other AI systems may begin to lose accuracy and become unreliable. The study was published in the esteemed journal Nature.
AI models function primarily as pattern-matching systems. They learn patterns in large datasets and use these patterns to provide responses to prompts. For instance, if you ask an AI model for a snickerdoodle recipe, it will give you the most common recipe it has learned rather than a unique version.
The Issue of Common Data
AI models often gravitate towards the most common output in their training datasets. If an AI is trained on countless images of golden retrievers, for example, it may start to assume that most dogs are golden retrievers. Therefore, when asked to generate a picture of a dog, it will likely produce an image of a golden retriever.
This tendency becomes problematic when AI models train on content generated by other AI systems. With a proliferation of AI-generated content on the web, new models may start ingesting and training on this content, leading to a skewed understanding of the data and potentially perpetuating inaccuracies.
Why AI Content Can Be Problematic
The internet is increasingly flooded with AI-generated data and content. As a result, when new AI models use this data for training, they might adopt the biases and inaccuracies of the previous AI-generated content.
This feedback loop leads to the ‘model collapse’ scenario, where AI models progressively become less accurate and more homogenous, as they fail to recognize the true diversity of the data they are supposed to represent.
This phenomenon can affect various AI models, including language models and image generators. The issue is especially problematic for tasks requiring specific and unique outputs, where common data might not provide sufficient accuracy.
Diversifying Training Data
To combat this issue, researchers emphasize the importance of diverse and high-quality training data. Ensuring that AI models are exposed to a wide range of data sources can help maintain their accuracy and reliability.
However, developing standardized benchmarks for data variety and ensuring qualitative and quantitative data sourcing remains a challenge. Meanwhile, solutions like watermarking AI-generated data could help models avoid reusing such data, although practical implementation is still lacking.
Corporate Concerns and Competition
Some companies might be reluctant to share their exclusive datasets, seeing them as a competitive advantage. Known as the ‘first mover advantage,’ companies may hoard original and human-generated data to maintain the superiority of their AI models.
This lack of data sharing can create disparities in AI capabilities across different organizations and exacerbate the problem of model collapse by limiting access to diverse datasets crucial for high-quality AI training.
To sustain the benefits of large-scale web-scraped data, it is essential for companies to prioritize the ethical use and sharing of valuable human interaction data, thus ensuring the development of robust AI models.
Mitigation Methods
Several methods have been proposed to mitigate the issue of model collapse. For example, benchmarks for qualitative and quantitative data sourcing can help enhance the quality of training datasets.
Additionally, implementing watermarking techniques for AI-generated data could prevent other models from inadvertently training on such data.
However, these mitigation methods require widespread adoption and cooperation across the AI industry to be effective. Without industry-wide standardization, the risk of model collapse may remain significant.
Future Implications
The threat of model collapse poses significant challenges for the future of AI development. Innovators must account for these risks to sustain the improvements and benefits brought by AI technologies.
As highlighted by the research, maintaining a broad and diverse range of high-quality training data is vital for the continued progress of AI. By addressing these issues head-on, the AI community can work towards creating more reliable and robust systems in the future.
The emergence of model collapse is a serious concern for the AI community, emphasizing the need for diverse and high-quality training data.
Addressing these challenges requires collaboration and innovative solutions to ensure AI models remain accurate and efficient. A concerted effort can help mitigate the threat and harness the full potential of AI technology.