The Building Blocks of Technological Evolution
3 min read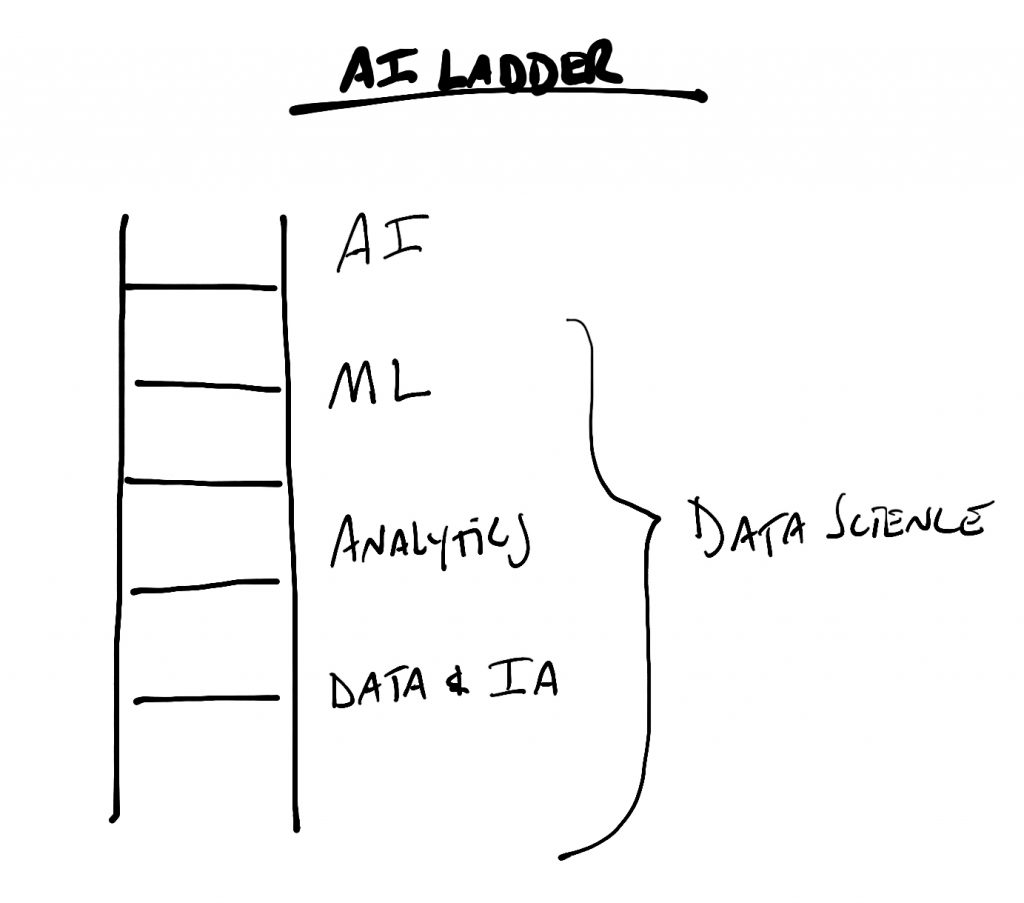
In 1890, the streets of Detroit witnessed the first automobile. However, it took another three decades for Henry Ford to make cars accessible to the masses. This story isn’t just about innovation; it’s about the crucial building blocks that make such advancements possible.
The history of automobiles teaches us a profound lesson. Initially, every single part had to be handcrafted, producing unique models like the Cugnot Steam Trolley. But once key components became standardized, mass production—and by extension, widespread adoption—suddenly became achievable. This process laid the groundwork for today’s technological marvels.
The Importance of Building Blocks in Innovation
In the early days of the automobile, every part had to be custom-made. This slow, bespoke approach resulted in unique creations like the Cugnot Steam Trolley. However, once standard components such as the combustion engine and the steering wheel were established, mass production became possible.
Over time, these building blocks enabled not just the scale and wider adoption of technology, but also drove complementary innovations. These new innovations in turn helped to refine and improve the original building blocks. This cycle of improvement and innovation is key to the success of any technological advancement.
AI: Following the Footsteps of Automobile Evolution
Much like the automobile industry, artificial intelligence (AI) relies on essential building blocks to thrive. AI needs machine learning, machine learning needs analytics, and analytics require a solid information architecture. In short, there is no AI without IA.
These capabilities form the solid rungs of the AI Ladder, a conceptual model describing the stages of analytic sophistication required to foster a productive AI environment. Mimicking and improving human functions, AI has the potential to revolutionize both daily life and enterprise operations.
Enterprise AI: The Real Challenge
“Enterprise AI” focuses on solving complex business problems in dynamic settings. This demands a clear understanding of unique use cases and starting points. As per Erik Brynjolfsson, the real hurdles are management, implementation, and business imagination.
It is crucial to recognize that each organization will have different entry points for AI. Some may leap to advanced AI technologies for specific tasks, while others need to first establish a solid information architecture.
To navigate these challenges, companies need to focus on three core areas: Hybrid Data Management, Unified Governance & Integration, and Data Science & Business Analytics.
Hybrid Data Management
Hybrid Data Management offers a unified platform to handle all types of data, irrespective of the source or destination. It brings together various data management techniques like SQL, NoSQL, row store, column store, and more.
An effective data strategy begins with hybrid data management, making it a singular approach for diverse data needs. By optimizing data through rich SQL access, organizations can streamline their operations and drive insights more effectively.
Unified Governance & Integration
Unified Governance & Integration ensures that data is accessible and secure across different cloud platforms. It involves robust data preparation, policy creation, authorization, auditing, and more.
With these components in place, organizations can prepare data efficiently and ensure it meets governance and security requirements. This makes data readily available for further analysis, providing a seamless experience.
Data Science & Business Analytics
Data Science & Business Analytics enable comprehensive and collaborative examination of all data types. These fields empower professionals to interpret complex data sets and extract meaningful insights economically.
Key components include analytics tools, advanced statistical models, and machine learning. This combination ensures that businesses can make data-driven decisions to enhance their performance.
With digitization and data explosion, the impact of machine learning on data has been transformative. However, there is still a need for a robust infrastructure to fully realize the potential of AI.
The Path Forward: Embracing AI
Adopting hybrid data management, unified governance & integration, and data science & business analytics is essential for leveraging AI effectively. This multi-faceted approach allows organizations of all sizes to unlock the power of AI.
By embracing these foundational technologies, companies can overcome the challenges of AI adoption and drive their business forward.
The evolution of automobiles and AI illustrates the power of foundational elements.
standardized components in cars and essential building blocks in AI both enable widespread adoption and innovation.
By understanding and leveraging these core principles, we can pave the way for future technological advancements.