This Week in AI: Companies Growing Skeptical of AI’s ROI
3 min read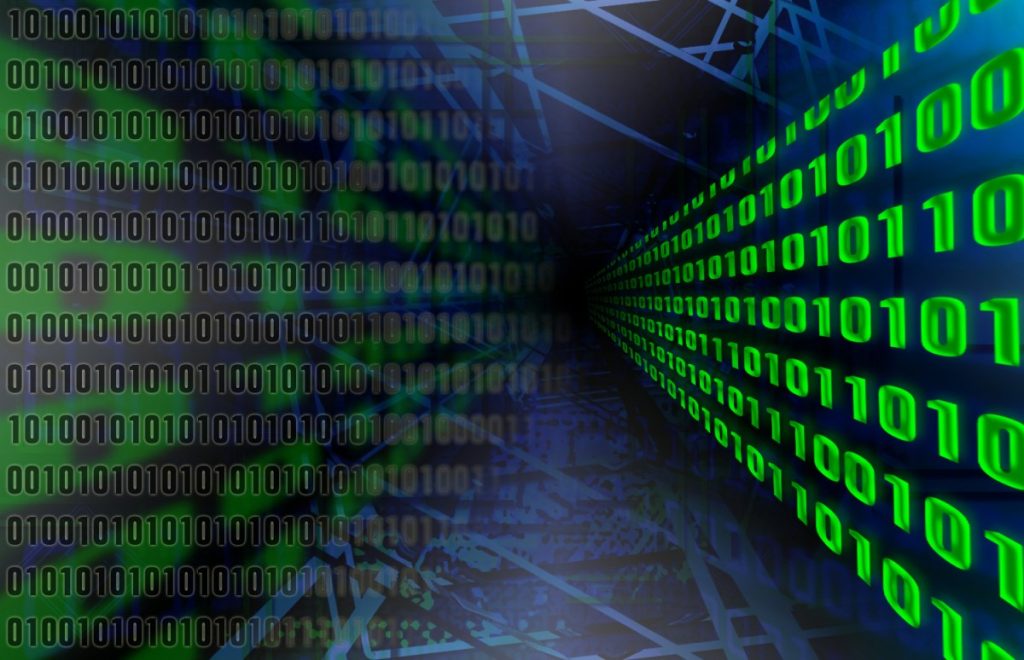
In the ever-evolving world of technology, artificial intelligence (AI) has garnered significant attention. However, recent trends suggest a growing skepticism among companies regarding AI’s return on investment (ROI).
This week, we delve into the reasons behind this shift in perception and the potential impact on the future of AI adoption in the corporate world.
Generative AI’s Uncertain Business Value
A recent report by Gartner indicates that around one-third of generative AI projects in enterprises will be abandoned after the proof-of-concept phase by the end of 2025. The primary reasons include poor data quality, inadequate risk controls, and escalating infrastructure costs.
One of the most significant challenges is the unclear business value of generative AI. Embracing this technology organization-wide can cost between $5 million and $20 million. For instance, a simple coding assistant has an upfront cost of $100,000 to $200,000, while an AI-powered document search tool can cost up to $11 million per user annually.
Productivity Paradox
A recent survey by Upwork highlights a paradox. Instead of enhancing productivity, AI has become a burden for many workers. Of the 2,500 C-suite executives, full-time staffers, and freelancers interviewed, nearly half (47%) reported that they have no idea how to achieve the productivity gains expected by their employers.
Furthermore, over three-fourths (77%) of workers believe that AI tools have decreased productivity and added to their workload. Anecdotal evidence supports this, with generative AI still plagued by fundamental technical issues.
Real-World Challenges
Bloomberg recently reported on a Google-powered tool used to analyze patient medical records at HCA hospitals in Florida. Unfortunately, this tool couldn’t consistently deliver reliable health information.
In one instance, it failed to note whether a patient had drug allergies. These stories are becoming increasingly common, highlighting the practical challenges and limitations of generative AI in real-world applications.
Companies are beginning to expect more from AI. Without significant research breakthroughs, vendors must manage expectations and be honest about the current limitations of AI.
Activity in the VC Arena
Despite growing skepticism, the venture capital (VC) sector remains active. AI startups continue to receive substantial funding. For example, Stability AI unveiled a generative AI model, Stable Video 4D, capable of turning a video of an object into multiple clips from different angles.
This model could revolutionize game development, video editing, and virtual reality. Stability AI is refining the model to handle a wider range of real-world videos beyond the current synthetic datasets.
The question remains whether these investments will yield the expected returns, given the lingering concerns about AI’s practicality and reliability.
Regulatory Frameworks and Ethical Concerns
The European Union (EU) has initiated a consultation on rules for providers of general-purpose AI models under the bloc’s AI Act. This risk-based framework aims to regulate AI applications and ensure they meet specific ethical and safety standards.
In the US, the Commerce Department recently endorsed “open-weight” generative AI models like Meta’s Llama 3.1. However, they recommend developing new capabilities to monitor these models for potential risks.
These regulatory efforts reflect a growing recognition of the need to balance innovation with ethical considerations to mitigate potential risks associated with AI.
Innovations and Progress
OpenAI is exploring alternatives to the traditional reinforcement learning from human feedback (RLHF) technique. In a new paper, researchers describe rule-based rewards (RBRs), which use step-by-step rules to evaluate and guide a model’s responses to prompts.
OpenAI claims that RBR-trained models demonstrate better safety performance and require less human feedback data. Since the launch of GPT-4, RBRs have been part of OpenAI’s safety stack, with plans to implement them in future models.
Such innovations could address some of the current limitations of AI, paving the way for safer and more reliable AI systems.
Breakthroughs in Complex Problem Solving
Google’s DeepMind has made significant strides in tackling complex math problems with AI. Two AI systems, AlphaProof and AlphaGeometry 2, solved four out of six problems in this year’s International Mathematical Olympiad (IMO).
While it took days to solve some problems and they struggled with open-ended questions, the results are promising. AlphaProof and AlphaGeometry 2 demonstrated abilities in abstraction and complex planning.
These achievements mark a significant step forward in the capabilities of AI systems, offering a glimpse into their potential in solving real-world problems.
The skepticism surrounding AI’s ROI is growing, but significant advancements and active investment in the sector indicate a complex landscape.
As companies and regulators grapple with ethical concerns and practical challenges, the future of AI remains both promising and uncertain.