Unlocking the Full Potential of Transformers Chain of Thought and Beyond
4 min read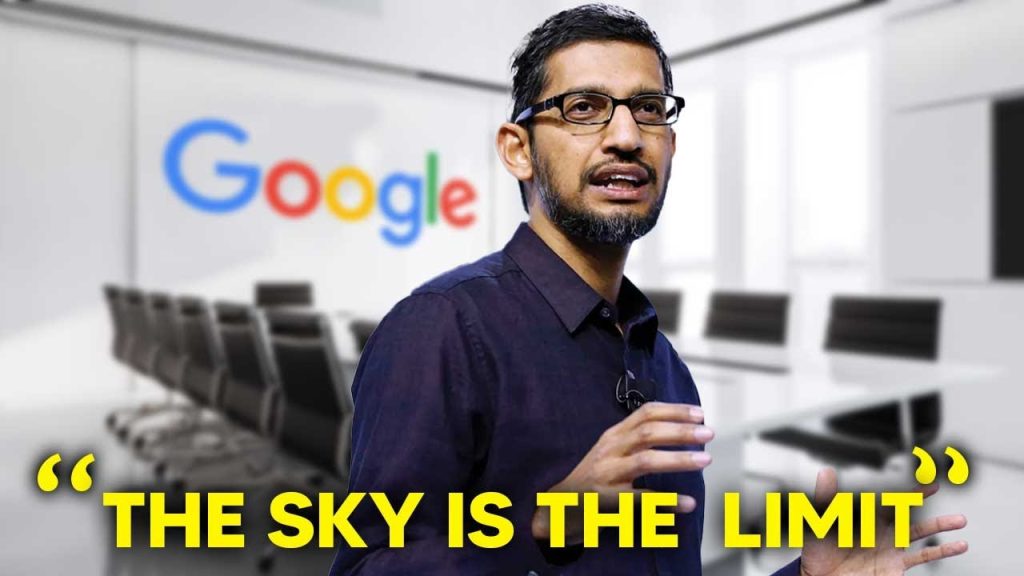
Today brought a groundbreaking revelation about the capabilities of Transformers from Google DeepMind.
Mathematically proving that Transformers can solve any problem with enough intermediate steps changes the landscape of AI. It highlights their potential beyond conventional limits.
Transformers: Unraveling the Mystery
Transformers have revolutionized modern AI by handling vast amounts of data simultaneously. This parallel processing makes them formidable text generators and language processors. However, their design struggles with tasks demanding sequential thinking, leading to limitations in solving step-by-step problems.
Chain of Thought (CoT) prompting emerges as the game-changer. It’s like asking a friend not just for an opinion, but for the reasoning behind it. This approach lets AI process information step-by-step, much like human reasoning, enhancing problem-solving capabilities.
Chain of Thought Prompting in Action
Imagine you’re planning a birthday party and ask a friend for ideas. Instead of a flat recommendation, with CoT, the friend walks you through their thinking process. This detailed reasoning provides valuable insights into each step, making the final suggestion more logical and tailored to your needs.
This mirrors how CoT works in AI. By breaking down complex problems into smaller, understandable steps, AI can tackle tricky issues more effectively. It’s like giving the AI a blueprint to follow, ensuring each part is well thought out.
Transformers’ Limitations and Chain of Thought Solutions
Transformers excel in parallel processing but falter with sequential tasks. For instance, a complex math problem needing multiple steps can trip them up. They tend to look at everything simultaneously, which is great for some tasks, but not all.
Chain of Thought prompting helps overcome these limitations. By guiding AI to generate intermediate reasoning steps, we allow it to think in a more structured and human-like manner. This process is crucial for solving problems that require a logical, step-by-step approach.
This doesn’t mean immediate solutions but emphasizes a gradual build-up. Each step taken by the AI is based on the previous one, making the process dynamic and adaptable to different problems.
Groundbreaking Claims of Transformers’ Capabilities
The recent claims about Transformers’ capabilities suggest that they can solve any problem if allowed to generate enough intermediate reasoning steps. This positions Transformers as versatile computational models, not just specialized text processors.
Transformers, when configured correctly, could emulate general-purpose computers. This means they are not limited to language tasks but could theoretically handle any computational domain.
This bold claim underscores the importance of generating intermediate reasoning tokens. These tokens represent the AI’s thought process, helping build up complex solutions iteratively.
Intermediate Reasoning Tokens: The Heart of CoT
Intermediate reasoning tokens are crucial for complex problem-solving. Like humans breaking down a difficult puzzle into manageable pieces, Transformers use these tokens to build a comprehensive solution.
Each token represents a step in the reasoning process. This dynamic approach allows the AI to adapt and construct its reasoning path as needed. It’s about guiding the model to think step by step.
By outputting a series of tokens, the AI provides a clear pathway of its decision-making process. This makes the reasoning transparent and easier to understand, expanding the types of problems AI can tackle.
Constant Depth Sufficiency: A Radical Concept
Typically, deeper neural networks are preferred for complex tasks due to their capacity to learn intricate data representations. However, this comes at a cost of increased computational resources and complexity.
The concept of constant depth sufficiency challenges this norm. It suggests that maintaining a fixed number of layers, while allowing for numerous intermediate steps, can achieve similar outcomes.
This approach reduces the need for deeper models, emphasizing efficient problem-solving through dynamic step generation. It simplifies the design, training, and deployment of AI models.
Implications for AI Development
This new understanding reshapes AI development strategies. Instead of pushing for deeper models, the focus shifts to enhancing intermediate step generation.
A shallow model with robust reasoning steps can outperform a deeper one by efficiently tackling sequential problems. This not only enhances performance but also reduces computational demands.
AI development can now prioritize versatile, efficient models capable of handling a wide range of tasks without the overhead of deeper architectures. This shift makes AI more accessible and practical.
Comparisons with OpenAI’s Model
OpenAI’s recent model validates the power of intermediate reasoning. It excels in complex challenges by thinking step-by-step, much like human reasoning.
This approach, demonstrated in competitive programming and science challenges, showcases the practical application of CoT principles. Both OpenAI and the recent Transformer studies highlight the shift towards better reasoning over sheer model size.
The emphasis is on training models to break down problems efficiently. It’s about smarter, not just bigger, AI.
Transformers and the Future of AI
The advancements in Transformer capabilities hint at a future where AI is more adaptable and powerful.
By embracing the Chain of Thought mechanism, we can unlock new potentials in problem-solving and decision-making.
The future of AI lies in models that think deeper, reason better, and solve complex problems with a strategic approach.
In summary, these insights into Transformers’ capabilities revolutionize our approach to AI models.
Moving from deeper layers to intermediate steps offers a more efficient and versatile way to tackle complex problems.
This shift promises a future of AI that is both powerful and practical, capable of solving a broader range of tasks.