Visual AI Models: More Hype Than Vision
5 min read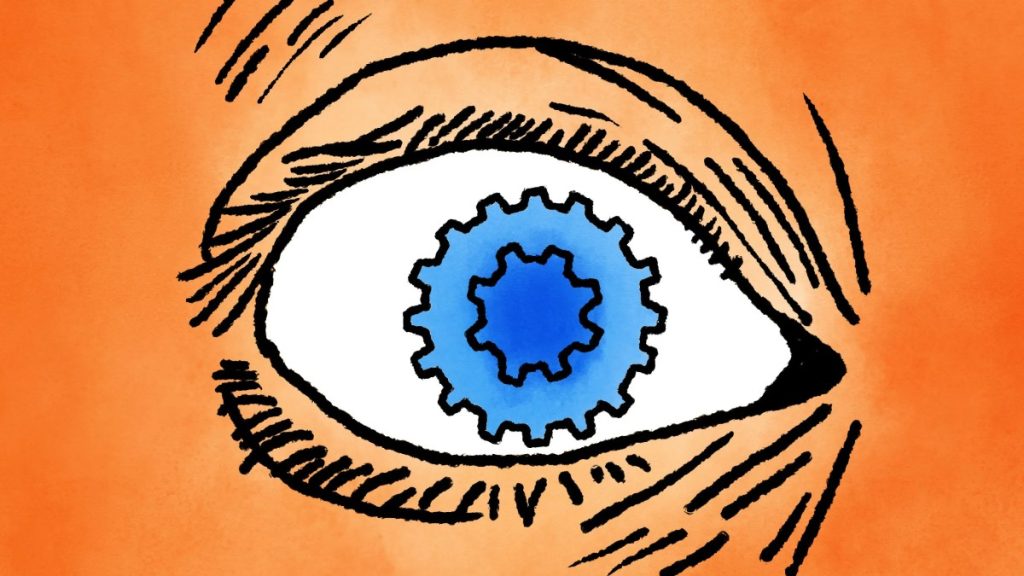
The latest AI models are promoted as advanced systems capable of understanding images, audio, and text seamlessly. However, a closer look reveals something startling: they don’t truly ‘see’ in the way humans do. This discrepancy between marketed capabilities and actual performance raises important questions about the effectiveness of these AI systems.
These models, marketed with terms like ‘vision capabilities’ and ‘visual understanding,’ aim to convince users they can handle visual data as effectively as textual data. They claim to solve various tasks, such as analyzing sports or helping with homework, using supposed visual prowess. However, in reality, these models merely match input patterns with their training data, leading to significant limitations in their true visual abilities.
Introduction to Visual AI Models
The latest AI models like GPT-4o and Gemini 1.5 Pro are praised for understanding images, audio, and text. However, a recent study reveals a stark truth: they don’t really ‘see’ like humans do. Although nobody has outright claimed that these models see like people, the marketing is misleading. Terms like ‘vision capabilities’ and ‘visual understanding’ suggest otherwise.
These AI models attempt to convince users that they can analyze images and videos just as adeptly as they handle text. Their touted abilities range from solving homework problems to watching sports. While these claims are intricately worded, it is evident that companies want to convey an impression of visual prowess. In reality, these models merely match patterns in the input data with patterns in their training data.
Exposing the Limitations
A group of researchers from Auburn University and the University of Alberta conducted a systematic and informal study to examine AI models’ visual understanding. They subjected the largest multimodal models to very simple visual tasks. These tasks included checking if two shapes overlap, counting pentagons, or identifying a circled letter in a word.
Shockingly, these tasks proved extremely difficult for the AI models. According to co-author Anh Nguyen, these tasks are simple enough for even a first-grader. Yet, the AI models struggled immensely, with error margins that shouldn’t exist for such straightforward tasks. Nguyen emphasized that if the best models are failing at these tasks, there’s a fundamental flaw.
Case Study: Overlapping Shapes
The overlapping shapes test showcased one of the simplest visual reasoning tasks possible. When presented with two circles either slightly overlapping, just touching, or with some distance between them, the models struggled. GPT-4o managed to get it right over 95% of the time when the circles were far apart. However, at zero or small distances, its accuracy plummeted to 18%.
Even though Gemini Pro 1.5 performed better, it only managed to get 7 out of 10 correct at close distances. The study thus pointed out the inconsistency in the models’ performance across different conditions. These inconsistencies are troubling, highlighting that what the models are doing doesn’t align with our notion of ‘seeing.’
Counting Circles and the Olympic Rings
Another test involved counting interlocking circles in an image. The AI models performed well when there were five rings, likely due to the Olympic Rings being prominently featured in their training data. However, they faltered miserably when an additional ring was added.
For instance, Gemini struggled and couldn’t get it right even once. Sonnet-3.5 only got it right a third of the time, while GPT-4o managed slightly better but still failed over half the time. Adding more rings further confused the models. This demonstrates that these models don’t ‘see’ as we do. Their perception is heavily influenced by the data they have been trained on.
This gap between their understanding and actual visual perception is underscored when the models do well on five-ring images due to their association with the Olympic Rings, yet fail on six- or seven-ring images. They haven’t learned to visually understand images beyond what’s in their training set.
The Concept of Blindness in AI Models
The term ‘blindness’ is apt when describing AI models’ inability to ‘see’ in a human sense. The AI models might extract approximate, abstract information from an image, like identifying a circle on the left side. However, they lack the ability to make nuanced visual judgments.
Co-author Anh Nguyen notes, “There is no existing technology that can visualize exactly what a model is seeing.” This leads to complex behaviors that combine input text prompts, image data, and model weights in unforeseen ways.
In one example, the model was asked about two overlapping circles and the resulting cyan-shaded area. A sighted person would easily identify this, but the AI model’s response was akin to an informed guess with eyes closed. This underscores the AI’s reliance on trained data over actual visual insight.
Misleading Marketing and Real Capabilities
Despite these limitations, these ‘visual’ AI models aren’t entirely useless. They excel in specific contexts, such as identifying human actions, expressions, and common objects in photos. Their intended purpose is to interpret such data accurately.
The marketing for these AI models, however, paints a misleading picture. It suggests they possess human-like visual abilities, which they clearly do not. Research is crucial to demystify these claims and showcase the models’ true capabilities.
This research sheds light on how these models operate. They are adept at recognizing familiar patterns but falter when asked to analyze unfamiliar visuals. Therefore, while they can tell if someone is sitting or walking, it’s not through ‘seeing’ as humans understand it.
Future of Visual AI Research
Looking ahead, continued research is essential to improving the visual understanding of AI models. This will involve not only refining their training data but also developing new methods to gauge their visual reasoning abilities.
As our reliance on AI grows, it’s important to have a clear understanding of their limitations and strengths. While they are powerful tools in many respects, expecting them to ‘see’ like humans is currently unrealistic.
Final Thoughts
This examination of ‘visual’ AI models reveals significant shortcomings in their ability to process visual information. They serve specific functions well but cannot replicate human vision. Future research will hopefully bridge this gap, improving their overall utility.
This deep dive into ‘visual’ AI models has revealed substantial limitations in their ability to handle visual data akin to human perception. While these models excel in specific applications, such as recognizing common objects or actions, they fall short in more nuanced visual tasks. Therefore, expecting these AI systems to ‘see’ like humans is currently unrealistic.
The findings underscore the need for continued research to improve these models’ visual understanding. The hope is to bridge the existing gap between their marketed capabilities and their actual performance. This will help in developing AI tools that are more reliable and effective in various contexts.